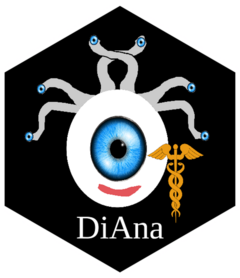
Disproportionality Analysis
Source:vignettes/articles/Disproportionality_Analysis.Rmd
Disproportionality_Analysis.Rmd
This tutorial will show you how to perform simple and advanced disproportionality analyses. While this tutorial recursively redefine the object of study and the background reference group, it does so to provide a pedagogical flow. It is recommended to design the analysis a priori, and clearly document and justify any deviation from the original design.
Requisites
This tutorial requires that you have first:
installed R and R Studio,
installed the DiAna package,
and set up your project folder.
Otherwise get back to the ReadMe and follow the instructions.
It is also recommended, but not necessary, that you have read the previous vignettes.
Start the subproject
From the DiAna main project, we open a new R script and run the snippet ‘new_FAERS_project’ to set up a project.
# Information -----------------------------------------------------------------
## Project title --------------------------------------------------------------
### v02_Disproportionality analysis
## Data -----------------------------------------------------------------------
### FDA Adverse Event Reporting System Quarterly Data
## Authors --------------------------------------------------------------------
### Michele Fusaroli
## Version --------------------------------------------------------------------
### Set up: 2024-07-29
### Last update: 2024-07-29
### DiAna version: 2.1.0
# Set up ----------------------------------------------------------------------
## upload DiAna ---------------------------------------------------------------
library(DiAna)
## Project_path ---------------------------------------------------------------
DiAna_path <- here::here()
project_name <- "v02_Disproportionality_Analysis"
project_path <- paste0(DiAna_path, "/projects/", project_name)
if (!file.exists(project_path)) {
dir.create(project_path)
}
project_path <- paste0(project_path, "/")
## FAERS_version --------------------------------------------------------------
FAERS_version <- "24Q1"
## Import data ----------------------------------------------------------------
import("DRUG")
#> primaryid drug_seq substance role_cod
#> <num> <int> <fctr> <ord>
#> 1: 4261825 1004486228 atropine C
#> 2: 4261825 1004486228 diphenoxylate C
#> 3: 4262057 1004487206 amoxicillin PS
#> 4: 4262057 1004487206 clavulanic acid PS
#> 5: 4262057 1004487210 macrogol C
#> ---
#> 69763501: 992001227 580 loratadine SS
#> 69763502: 992001227 581 loratadine SS
#> 69763503: 992001227 582 loratadine SS
#> 69763504: 992001227 586 magnesium C
#> 69763505: 992001227 587 magnesium C
import("REAC")
#> primaryid pt drug_rec_act
#> <num> <ord> <ord>
#> 1: 4204616 abdominal pain <NA>
#> 2: 4204616 heart rate increased <NA>
#> 3: 4204616 nausea <NA>
#> 4: 4204616 pyrexia <NA>
#> 5: 4204616 uterine tenderness <NA>
#> ---
#> 52222720: 237079291 chemical burn of skin <NA>
#> 52222721: 237079291 toxicity to various agents <NA>
#> 52222722: 237079291 scar <NA>
#> 52222723: 237203351 injection site discolouration <NA>
#> 52222724: 237203351 injection site bruising <NA>
## Input files ----------------------------------------------------------------
## Output files ---------------------------------------------------------------
## Definitions ----------------------------------------------------------------
## Reference groups -----------------------------------------------------------
## Descriptive analysis -------------------------------------------------------
## Disproportionality analysis ------------------------------------------------
## Case by case analysis ------------------------------------------------------
Disproportionality analysis
Basic application
Let’s explore the disproportionality_analysis function. We are investigating the possibility of a role of “aripiprazole” in causing “impulse control disorder” (ICD), and we therefore draft the definitions of our objects of study.
## Definitions --------------------------------------------------------------------------
drug_selected <- "aripiprazole"
reac_selected <- "impulse control disorder"
Already using these simple definitions, we can ran the simplest disproportionality analysis: we want to save in an object called disproportionality_df, the results of a disproportionality analysis on the drug and reaction specified.
## Disproportionality analysis ----------------------------------------------------------
disproportionality_df <- disproportionality_analysis(
drug_selected = drug_selected,
reac_selected = reac_selected
)
As you run the command a message appears in the console: “Not all the events selected were found in the database, check the following terms for any misspelling or alternative nomenclature: impulse control disorder. Would you like to revise the query? (Yes/no/cancel)”. This message implies that the exact term “impulse control disorder” does not exists in our database. How can we proceed? We write “Yes” in the console and run the command with “return”. The function warns us once again to revise our query, or definition of the object of study. How can we check the correct spelling? We can look into the MedDRA if we have it, or more quickly search directly in the database with the following command, that could be run just in the console since we do not need to keep it in our script.
pt | N |
---|---|
impulsive behaviour | 3264 |
impulse-control disorder | 1733 |
The previous command can be read as: “within the database Reac, count how many times (.N) each preferred term occurs (by=”pt”). Then order the pt by decreasing N (order(-N)). Finally, filter only the rows with the pattern “impuls” in the pt. As you can see there are two terms that we should plausibly include in out analysis: impulsive behaviour, which is the term most used even if we did not even consider it, and “impulse-control disorder” which is the term we wanted to consider but has also a dash between the first two words. First, let’s fix the definition. We get back to the definition section of our script and we fix it including the dash in the reaction pt as follows and we rerun the disproportionality analysis:
## Definitions --------------------------------------------------------------------------
drug_selected <- "aripiprazole"
reac_selected <- "impulse-control disorder"
## Disproportionality analysis ----------------------------------------------------------
disproportionality_df <- disproportionality_analysis(
drug_selected = drug_selected,
reac_selected = reac_selected
)
## Definitions --------------------------------------------------------------------------
disproportionality_df
substance | event | D_E | D_nE | D | nD_E | E | nD_nE | ROR_median | ROR_lower | ROR_upper | IC_median | IC_lower | IC_upper | label_ROR | label_IC | Bonferroni | ROR_signal | IC_signal |
---|---|---|---|---|---|---|---|---|---|---|---|---|---|---|---|---|---|---|
aripiprazole | impulse-control disorder | 286 | 111261 | 111547 | 1447 | 1733 | 17485485 | 31.06 | 27.28 | 35.25 | 4.64 | 4.44 | 4.78 | 31.06 (27.28-35.25) [286] | 4.64 (4.44-4.78) [286] | TRUE | SDR | SDR |
This time no error appears, and after few seconds we can see that our environment (window pane usually on the upper right) has been populated with a dataset called disproportionalitY_df. Calling the name of the object prints it in the console, and we can see that the object is now a dataset with one row (per drug-event combination) and 19 columns, including the name of the substance and the event, the instances of the contingency table, the median and 95% confidence interval of the Reporting Odds Ratio ROR and of the Information component, these same results summarised as a string in the following format “median (lower limit-upper limit) [N cases]”. A Bonferroni column that specifies whether the ROR was still significant after correcting for multiple comparison, and two columns that summarise whether a signal exists (SDR) or not (no SDR) or exists but disappear at the correction for multiple comparison (weak SDR). In this case we have found a signal of disproportionate reporting using both the analyses. These columns exist to simplify input to further functions, such as, for example, the visualization using render_forest.
render_forest(disproportionality_df, index = "IC")
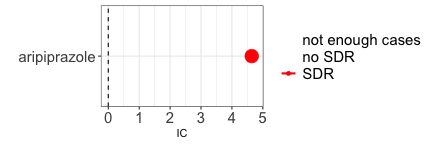
render_forest(disproportionality_df, index = "ROR")
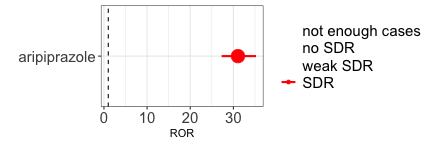
In these forest plots we see on the x axis the information component (by default) or the ROR if we specify it in the index parameter. The median is shown using the dot, whose size is proportional to the number of cases. The 95% confidence interval is shown by the line. A dashed vertical axis (on the 0 for the IC and on the 1 for the ROR) highlights the threshold of statistical significance, compared to which the lower limit of the confidence interval must be higher to identify a signal of disproportionate reporting. In this case, we identified a clear signal of disproportionate reporting using both methods.
Extend queries
As we said before, the definition is not exhaustive, and we risk missing many cases of impulsivity which used a different PT from “impulse-control disorder”. In fact those undetected cases will be part of the background reference group and potentially distort the results. How can we extend the definition? Let’s go back to the definition paragraph and add other interesting terms to the definition of impulse-control disorder, using a named list.
## Definitions --------------------------------------------------------------------------
drug_selected <- "aripiprazole"
reac_selected <- list("impulse-control disorder" = list(
"impulse-control disorder",
"impulsive behaviour"
))
## Disproportionality analysis ----------------------------------------------------------
disproportionality_df <- disproportionality_analysis(
drug_selected = drug_selected,
reac_selected = reac_selected
)
## Definitions --------------------------------------------------------------------------
disproportionality_df
substance | event | D_E | D_nE | D | nD_E | E | nD_nE | ROR_median | ROR_lower | ROR_upper | IC_median | IC_lower | IC_upper | label_ROR | label_IC | Bonferroni | ROR_signal | IC_signal |
---|---|---|---|---|---|---|---|---|---|---|---|---|---|---|---|---|---|---|
aripiprazole | impulse-control disorder | 701 | 110846 | 111547 | 4258 | 4959 | 17482674 | 25.94 | 23.94 | 28.13 | 4.45 | 4.33 | 4.54 | 25.94 (23.94-28.13) [701] | 4.45 (4.33-4.54) [701] | TRUE | SDR | SDR |
render_forest(disproportionality_df)
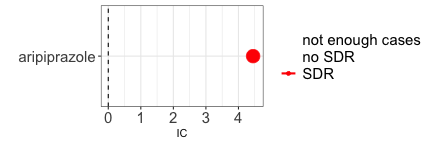
You see now that the number of cases has increased from 286 to 701, and we still find a SDR.
Multiple queries
Taking this further, we should consider that this impulsivity manifests often as pervasive behaviors, such as gambling, compulsive shopping, hyperphagia, and hypersexuality. Here below we add an extended query for impulse-control disorders that we refined over the years (see Parkinsonism and Related Disorders 90 (2021) 79–83 for the documentation of how we obtained the first definition combining a scoping review and disproportionality analysis). We also use a further feature of the disproportionality_analysis function and run the analysis in parallel on the several possible behavioral manifestations of impulsivity, just adding other named elements to the list.
Specifically, we first define a named list called ICDs with the subqueries related with the different manifestations (e.g., gambling disorder could be reported either as gambling disorder or as gambling), and we then run rlist append to store in the reac_selected parameter both the individual sub-queries and the full query. When we run the analysis we see again the message that some of the terms (“erotophonophilia” and “frotteurism” were not found, but in this case we know that these terms are spelled correctly (it is just the case that noone used them in the FAERS yet) and we run “no” in the console to let the analysis proceed.
In this case we also add a further parameter to the render_forest function, to make it print each event in a different line
## Definitions --------------------------------------------------------------------------
drug_selected <- "aripiprazole"
ICDs <- list(
"gambling disorder" = list("gambling disorder", "gambling"),
"hypersexuality" = list(
"compulsive sexual behaviour",
"hypersexuality",
"excessive masturbation",
"excessive sexual fantasies",
"libido increased",
"sexual activity increased",
"kluver-bucy syndrome"
),
"paraphilia" = list(
"erotophonophilia", "exhibitionism",
"fetishism", "frotteurism",
"masochism", "paraphilia", "paedophilia",
"sadism", "transvestism", "voyeurism",
"sexually inappropriate behaviour"
),
"compulsive shopping" = list("compulsive shopping"),
"hyperphagia" = list(
"binge eating", "food craving", "hyperphagia",
"increased appetite"
),
"gaming disorder" = list("gaming disorder"),
"pyromania" = list("pyromania"),
"kleptomania" = list("kleptomania", "shoplifting"),
"compulsive hoarding" = list("compulsive hoarding"),
"excessive exercise" = list("excessive exercise"),
"overwork" = list("overwork"),
"poriomania" = list("poriomania"),
"body-focused disorder" = list(
"compulsive cheek biting",
"compulsive lip biting",
"dermatillomania",
"dermatophagia", "nail picking",
"compulsive handwashing",
"trichotemnomania",
"trichotillomania", "onychophagia",
"thumb sucking"
),
"stereotypy" = list("automatism", "stereotypy"),
"impulsivity" = list(
"impulse-control disorder",
"impulsive behaviour",
"disinhibition", "behavioural addiction",
"abnormal behaviour"
)
)
reac_selected <- rlist::list.append(ICDs, "ICD" = as.list(unlist(purrr::flatten(ICDs))))
## Disproportionality analysis ----------------------------------------------------------
disproportionality_df <- disproportionality_analysis(
drug_selected = drug_selected,
reac_selected = reac_selected
)
## Definitions --------------------------------------------------------------------------
disproportionality_df
substance | event | D_E | D_nE | D | nD_E | E | nD_nE | ROR_median | ROR_lower | ROR_upper | IC_median | IC_lower | IC_upper | label_ROR | label_IC | Bonferroni | ROR_signal | IC_signal |
---|---|---|---|---|---|---|---|---|---|---|---|---|---|---|---|---|---|---|
aripiprazole | impulsivity | 2324 | 109223 | 111547 | 39764 | 42088 | 17447168 | 9.33 | 8.94 | 9.73 | 3.12 | 3.05 | 3.17 | 9.33 (8.94-9.73) [2324] | 3.12 (3.05-3.17) [2324] | TRUE | SDR | SDR |
aripiprazole | ICD | 5898 | 105649 | 111547 | 66398 | 72296 | 17420534 | 14.64 | 14.24 | 15.04 | 3.68 | 3.64 | 3.71 | 14.64 (14.24-15.04) [5898] | 3.68 (3.64-3.71) [5898] | TRUE | SDR | SDR |
aripiprazole | hyperphagia | 1114 | 110433 | 111547 | 20160 | 21274 | 17466772 | 8.73 | 8.21 | 9.28 | 3.04 | 2.94 | 3.11 | 8.73 (8.21-9.28) [1114] | 3.04 (2.94-3.11) [1114] | TRUE | SDR | SDR |
aripiprazole | gambling disorder | 2479 | 109068 | 111547 | 2589 | 5068 | 17484343 | 153.42 | 144.49 | 162.66 | 6.24 | 6.18 | 6.29 | 153.42 (144.49-162.66) [2479] | 6.24 (6.18-6.29) [2479] | TRUE | SDR | SDR |
aripiprazole | body-focused disorder | 200 | 111347 | 111547 | 1109 | 1309 | 17485823 | 28.33 | 24.23 | 32.98 | 4.51 | 4.27 | 4.67 | 28.33 (24.23-32.98) [200] | 4.51 (4.27-4.67) [200] | TRUE | SDR | SDR |
aripiprazole | hypersexuality | 1119 | 110428 | 111547 | 3077 | 4196 | 17483855 | 57.56 | 53.72 | 61.60 | 5.36 | 5.26 | 5.44 | 57.56 (53.72-61.6) [1119] | 5.36 (5.26-5.44) [1119] | TRUE | SDR | SDR |
aripiprazole | paraphilia | 92 | 111455 | 111547 | 511 | 603 | 17486421 | 28.25 | 22.37 | 35.30 | 4.41 | 4.07 | 4.66 | 28.25 (22.37-35.3) [92] | 4.41 (4.07-4.66) [92] | TRUE | SDR | SDR |
aripiprazole | kleptomania | 195 | 111352 | 111547 | 149 | 344 | 17486783 | 206.74 | 164.42 | 258.48 | 6.18 | 5.95 | 6.36 | 206.74 (164.42-258.48) [195] | 6.18 (5.95-6.36) [195] | TRUE | SDR | SDR |
aripiprazole | stereotypy | 60 | 111487 | 111547 | 942 | 1002 | 17485990 | 9.98 | 7.56 | 12.97 | 3.14 | 2.71 | 3.45 | 9.98 (7.56-12.97) [60] | 3.14 (2.71-3.45) [60] | TRUE | SDR | SDR |
aripiprazole | excessive exercise | 1 | 111546 | 111547 | 84 | 85 | 17486848 | 1.86 | 0.04 | 10.68 | 0.53 | -3.26 | 2.21 | 1.86 (0.04-10.68) [1] | 0.53 (-3.26-2.21) [1] | FALSE | not enough cases | not enough cases |
aripiprazole | pyromania | 6 | 111541 | 111547 | 91 | 97 | 17486841 | 10.33 | 3.69 | 23.36 | 2.54 | 1.12 | 3.45 | 10.33 (3.69-23.36) [6] | 2.54 (1.12-3.45) [6] | TRUE | SDR | SDR |
aripiprazole | poriomania | 16 | 111531 | 111547 | 157 | 173 | 17486775 | 15.97 | 8.91 | 26.77 | 3.36 | 2.52 | 3.95 | 15.97 (8.91-26.77) [16] | 3.36 (2.52-3.95) [16] | TRUE | SDR | SDR |
aripiprazole | overwork | 2 | 111545 | 111547 | 70 | 72 | 17486862 | 4.47 | 0.53 | 16.79 | 1.38 | -1.21 | 2.77 | 4.47 (0.53-16.79) [2] | 1.38 (-1.21-2.77) [2] | FALSE | not enough cases | not enough cases |
aripiprazole | compulsive hoarding | 245 | 111302 | 111547 | 75 | 320 | 17486857 | 511.88 | 396.39 | 674.49 | 6.60 | 6.39 | 6.75 | 511.88 (396.39-674.49) [245] | 6.6 (6.39-6.75) [245] | TRUE | SDR | SDR |
aripiprazole | compulsive shopping | 1108 | 110439 | 111547 | 782 | 1890 | 17486150 | 224.01 | 203.60 | 247.78 | 6.47 | 6.37 | 6.54 | 224.01 (203.6-247.78) [1108] | 6.47 (6.37-6.54) [1108] | TRUE | SDR | SDR |
aripiprazole | gaming disorder | 3 | 111544 | 111547 | 6 | 9 | 17486926 | 78.38 | 12.68 | 365.96 | 2.65 | 0.58 | 3.85 | 78.38 (12.68-365.96) [3] | 2.65 (0.58-3.85) [3] | TRUE | SDR | SDR |
render_forest(disproportionality_df, row = "event")
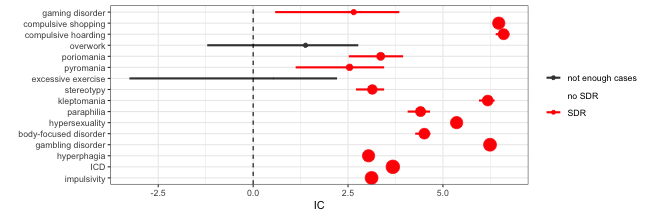
The results now show 16 rows (one per drug-event combination), for which we have found all SDR apart that for excessive exercise and overwork for which we have found less than 3 cases (the minimum to identify an SDR with the IC. We can see in the render forests how the confidence interval is larger for combinations in which there are fewer cases (compare with the different size of the dots).
Positive and negative controls
The same considerations can in fact be performed also on the drug (we may want to check the association with aripiprazole alone and with its pharmacological class as a group (third generation antipsychotics: also brexpiprazole and cariprazine), and can be used to include also negative and positive controls: we may include “pramipexole” as a positive control (a drug which is known to cause impulsivity), “paracetamol” as a negative control (a drug which is known to not cause impulsivity), and potentially also some events that are known reactions to aripiprazole (e.g., insomnia, headache) and some events that are known with good certainty to not be reactions to aripiprazole (“liver injury”).
In this case we may want to add to the render forest the option to give each its drug its facet, as seen in the script.
## Definitions --------------------------------------------------------------------------
drug_selected <- list(
"aripiprazole" = list("aripiprazole"),
"TGAs" = list("aripiprazole", "brexpiprazole", "cariprazine"),
"pramipexole" = list("pramipexole"), # positive control
"paracetamol" = list("paracetamol")
) # negative control
ICDs <- list(
"gambling disorder" = list("gambling disorder", "gambling"),
"hypersexuality" = list(
"compulsive sexual behaviour",
"hypersexuality",
"excessive masturbation",
"excessive sexual fantasies",
"libido increased",
"sexual activity increased",
"kluver-bucy syndrome"
),
"paraphilia" = list(
"erotophonophilia", "exhibitionism",
"fetishism", "frotteurism",
"masochism", "paraphilia", "paedophilia",
"sadism", "transvestism", "voyeurism",
"sexually inappropriate behaviour"
),
"compulsive shopping" = list("compulsive shopping"),
"hyperphagia" = list(
"binge eating", "food craving", "hyperphagia",
"increased appetite"
),
"gaming disorder" = list("gaming disorder"),
"pyromania" = list("pyromania"),
"kleptomania" = list("kleptomania", "shoplifting"),
"compulsive hoarding" = list("compulsive hoarding"),
"excessive exercise" = list("excessive exercise"),
"overwork" = list("overwork"),
"poriomania" = list("poriomania"),
"body-focused disorder" = list(
"compulsive cheek biting",
"compulsive lip biting",
"dermatillomania",
"dermatophagia", "nail picking",
"compulsive handwashing",
"trichotemnomania",
"trichotillomania", "onychophagia",
"thumb sucking"
),
"stereotypy" = list("automatism", "stereotypy"),
"impulsivity" = list(
"impulse-control disorder",
"impulsive behaviour",
"disinhibition", "behavioural addiction",
"abnormal behaviour"
)
)
reac_selected <- rlist::list.append(ICDs,
"ICD" = as.list(unlist(purrr::flatten(ICDs))),
"insomnia" = list("insomnia"), # positive control
"headache" = list("headache"), # positive control
"liver injury" = list("liver injury")
) # negative control
## Disproportionality analysis ----------------------------------------------------------
# Positive and negative controls
disproportionality_df <- disproportionality_analysis(
drug_selected = drug_selected,
reac_selected = reac_selected
)
## Definitions --------------------------------------------------------------------------
disproportionality_df
substance | event | D_E | D_nE | D | nD_E | E | nD_nE | ROR_median | ROR_lower | ROR_upper | IC_median | IC_lower | IC_upper | label_ROR | label_IC | Bonferroni | ROR_signal | IC_signal |
---|---|---|---|---|---|---|---|---|---|---|---|---|---|---|---|---|---|---|
paracetamol | headache | 42550 | 886402 | 928952 | 495075 | 537625 | 16174452 | 1.56 | 1.55 | 1.58 | 0.58 | 0.56 | 0.59 | 1.56 (1.55-1.58) [42550] | 0.58 (0.56-0.59) [42550] | TRUE | SDR | SDR |
TGAs | headache | 3297 | 125209 | 128506 | 534328 | 537625 | 16935645 | 0.83 | 0.80 | 0.86 | -0.26 | -0.31 | -0.22 | 0.83 (0.8-0.86) [3297] | -0.26 (-0.31–0.22) [3297] | FALSE | no SDR | no SDR |
aripiprazole | headache | 2901 | 108646 | 111547 | 534724 | 537625 | 16952208 | 0.84 | 0.81 | 0.87 | -0.24 | -0.30 | -0.19 | 0.84 (0.81-0.87) [2901] | -0.24 (-0.3–0.19) [2901] | FALSE | no SDR | no SDR |
pramipexole | headache | 1218 | 32344 | 33562 | 536407 | 537625 | 17028510 | 1.19 | 1.12 | 1.26 | 0.24 | 0.15 | 0.31 | 1.19 (1.12-1.26) [1218] | 0.24 (0.15-0.31) [1218] | TRUE | SDR | SDR |
paracetamol | insomnia | 18338 | 910614 | 928952 | 213916 | 232254 | 16455611 | 1.54 | 1.52 | 1.57 | 0.58 | 0.55 | 0.59 | 1.54 (1.52-1.57) [18338] | 0.58 (0.55-0.59) [18338] | TRUE | SDR | SDR |
TGAs | insomnia | 4158 | 124348 | 128506 | 228096 | 232254 | 17241877 | 2.52 | 2.44 | 2.60 | 1.29 | 1.24 | 1.33 | 2.52 (2.44-2.6) [4158] | 1.29 (1.24-1.33) [4158] | TRUE | SDR | SDR |
aripiprazole | insomnia | 3649 | 107898 | 111547 | 228605 | 232254 | 17258327 | 2.55 | 2.46 | 2.63 | 1.30 | 1.25 | 1.34 | 2.55 (2.46-2.63) [3649] | 1.3 (1.25-1.34) [3649] | TRUE | SDR | SDR |
pramipexole | insomnia | 1368 | 32194 | 33562 | 230886 | 232254 | 17334031 | 3.19 | 3.01 | 3.36 | 1.62 | 1.53 | 1.69 | 3.19 (3.01-3.36) [1368] | 1.62 (1.53-1.69) [1368] | TRUE | SDR | SDR |
paracetamol | impulsivity | 2670 | 926282 | 928952 | 39418 | 42088 | 16630109 | 1.21 | 1.16 | 1.26 | 0.26 | 0.20 | 0.31 | 1.21 (1.16-1.26) [2670] | 0.26 (0.2-0.31) [2670] | TRUE | SDR | SDR |
TGAs | impulsivity | 2463 | 126043 | 128506 | 39625 | 42088 | 17430348 | 8.59 | 8.24 | 8.95 | 3.00 | 2.93 | 3.04 | 8.59 (8.24-8.95) [2463] | 3 (2.93-3.04) [2463] | TRUE | SDR | SDR |
aripiprazole | impulsivity | 2324 | 109223 | 111547 | 39764 | 42088 | 17447168 | 9.33 | 8.94 | 9.73 | 3.12 | 3.05 | 3.17 | 9.33 (8.94-9.73) [2324] | 3.12 (3.05-3.17) [2324] | TRUE | SDR | SDR |
pramipexole | impulsivity | 846 | 32716 | 33562 | 41242 | 42088 | 17523675 | 10.98 | 10.24 | 11.77 | 3.38 | 3.27 | 3.47 | 10.98 (10.24-11.77) [846] | 3.38 (3.27-3.47) [846] | TRUE | SDR | SDR |
paracetamol | ICD | 4319 | 924633 | 928952 | 67977 | 72296 | 16601550 | 1.14 | 1.10 | 1.17 | 0.17 | 0.12 | 0.21 | 1.14 (1.1-1.17) [4319] | 0.17 (0.12-0.21) [4319] | TRUE | SDR | SDR |
TGAs | ICD | 6379 | 122127 | 128506 | 65917 | 72296 | 17404056 | 13.79 | 13.42 | 14.16 | 3.59 | 3.55 | 3.62 | 13.79 (13.42-14.16) [6379] | 3.59 (3.55-3.62) [6379] | TRUE | SDR | SDR |
aripiprazole | ICD | 5898 | 105649 | 111547 | 66398 | 72296 | 17420534 | 14.64 | 14.24 | 15.04 | 3.68 | 3.64 | 3.71 | 14.64 (14.24-15.04) [5898] | 3.68 (3.64-3.71) [5898] | TRUE | SDR | SDR |
pramipexole | ICD | 2669 | 30893 | 33562 | 69627 | 72296 | 17495290 | 21.70 | 20.84 | 22.61 | 4.26 | 4.20 | 4.31 | 21.7 (20.84-22.61) [2669] | 4.26 (4.2-4.31) [2669] | TRUE | SDR | SDR |
paracetamol | hyperphagia | 1170 | 927782 | 928952 | 20104 | 21274 | 16649423 | 1.04 | 0.98 | 1.10 | 0.05 | -0.04 | 0.12 | 1.04 (0.98-1.1) [1170] | 0.05 (-0.04-0.12) [1170] | FALSE | no SDR | no SDR |
TGAs | hyperphagia | 1310 | 127196 | 128506 | 19964 | 21274 | 17450009 | 9.00 | 8.50 | 9.52 | 3.07 | 2.98 | 3.13 | 9 (8.5-9.52) [1310] | 3.07 (2.98-3.13) [1310] | TRUE | SDR | SDR |
aripiprazole | hyperphagia | 1114 | 110433 | 111547 | 20160 | 21274 | 17466772 | 8.73 | 8.21 | 9.28 | 3.04 | 2.94 | 3.11 | 8.73 (8.21-9.28) [1114] | 3.04 (2.94-3.11) [1114] | TRUE | SDR | SDR |
pramipexole | hyperphagia | 346 | 33216 | 33562 | 20928 | 21274 | 17543989 | 8.73 | 7.82 | 9.71 | 3.07 | 2.89 | 3.20 | 8.73 (7.82-9.71) [346] | 3.07 (2.89-3.2) [346] | TRUE | SDR | SDR |
paracetamol | gambling disorder | 311 | 928641 | 928952 | 4757 | 5068 | 16664770 | 1.17 | 1.04 | 1.31 | 0.21 | 0.02 | 0.35 | 1.17 (1.04-1.31) [311] | 0.21 (0.02-0.35) [311] | FALSE | SDR | SDR |
TGAs | gambling disorder | 2568 | 125938 | 128506 | 2500 | 5068 | 17467473 | 142.23 | 135.00 | 151.42 | 6.09 | 6.03 | 6.14 | 142.23 (135-151.42) [2568] | 6.09 (6.03-6.14) [2568] | TRUE | SDR | SDR |
aripiprazole | gambling disorder | 2479 | 109068 | 111547 | 2589 | 5068 | 17484343 | 153.42 | 144.49 | 162.66 | 6.24 | 6.18 | 6.29 | 153.42 (144.49-162.66) [2479] | 6.24 (6.18-6.29) [2479] | TRUE | SDR | SDR |
pramipexole | gambling disorder | 1593 | 31969 | 33562 | 3475 | 5068 | 17561442 | 252.51 | 237.49 | 270.92 | 7.29 | 7.20 | 7.35 | 252.51 (237.49-270.92) [1593] | 7.29 (7.2-7.35) [1593] | TRUE | SDR | SDR |
paracetamol | body-focused disorder | 74 | 928878 | 928952 | 1235 | 1309 | 16668292 | 1.07 | 0.83 | 1.36 | 0.09 | -0.29 | 0.37 | 1.07 (0.83-1.36) [74] | 0.09 (-0.29-0.37) [74] | FALSE | no SDR | no SDR |
TGAs | body-focused disorder | 207 | 128299 | 128506 | 1102 | 1309 | 17468871 | 25.55 | 21.93 | 29.71 | 4.36 | 4.13 | 4.53 | 25.55 (21.93-29.71) [207] | 4.36 (4.13-4.53) [207] | TRUE | SDR | SDR |
aripiprazole | body-focused disorder | 200 | 111347 | 111547 | 1109 | 1309 | 17485823 | 28.33 | 24.23 | 32.98 | 4.51 | 4.27 | 4.67 | 28.33 (24.23-32.98) [200] | 4.51 (4.27-4.67) [200] | TRUE | SDR | SDR |
pramipexole | body-focused disorder | 16 | 33546 | 33562 | 1293 | 1309 | 17563624 | 6.47 | 3.69 | 10.56 | 2.46 | 1.61 | 3.04 | 6.47 (3.69-10.56) [16] | 2.46 (1.61-3.04) [16] | TRUE | SDR | SDR |
paracetamol | hypersexuality | 216 | 928736 | 928952 | 3980 | 4196 | 16665547 | 0.97 | 0.84 | 1.11 | -0.04 | -0.27 | 0.12 | 0.97 (0.84-1.11) [216] | -0.04 (-0.27-0.12) [216] | FALSE | no SDR | no SDR |
TGAs | hypersexuality | 1161 | 127345 | 128506 | 3035 | 4196 | 17466938 | 52.53 | 49.01 | 56.26 | 5.22 | 5.12 | 5.29 | 52.53 (49.01-56.26) [1161] | 5.22 (5.12-5.29) [1161] | TRUE | SDR | SDR |
aripiprazole | hypersexuality | 1119 | 110428 | 111547 | 3077 | 4196 | 17483855 | 57.56 | 53.72 | 61.60 | 5.36 | 5.26 | 5.44 | 57.56 (53.72-61.6) [1119] | 5.36 (5.26-5.44) [1119] | TRUE | SDR | SDR |
pramipexole | hypersexuality | 584 | 32978 | 33562 | 3612 | 4196 | 17561305 | 86.13 | 78.75 | 93.91 | 6.10 | 5.96 | 6.20 | 86.13 (78.75-93.91) [584] | 6.1 (5.96-6.2) [584] | TRUE | SDR | SDR |
paracetamol | paraphilia | 22 | 928930 | 928952 | 581 | 603 | 16668946 | 0.67 | 0.42 | 1.03 | -0.53 | -1.24 | -0.03 | 0.67 (0.42-1.03) [22] | -0.53 (-1.24–0.03) [22] | FALSE | no SDR | no SDR |
TGAs | paraphilia | 95 | 128411 | 128506 | 508 | 603 | 17469465 | 25.42 | 20.20 | 31.75 | 4.28 | 3.94 | 4.52 | 25.42 (20.2-31.75) [95] | 4.28 (3.94-4.52) [95] | TRUE | SDR | SDR |
aripiprazole | paraphilia | 92 | 111455 | 111547 | 511 | 603 | 17486421 | 28.25 | 22.37 | 35.30 | 4.41 | 4.07 | 4.66 | 28.25 (22.37-35.3) [92] | 4.41 (4.07-4.66) [92] | TRUE | SDR | SDR |
pramipexole | paraphilia | 62 | 33500 | 33562 | 541 | 603 | 17564376 | 60.09 | 45.36 | 78.41 | 5.24 | 4.82 | 5.54 | 60.09 (45.36-78.41) [62] | 5.24 (4.82-5.54) [62] | TRUE | SDR | SDR |
paracetamol | kleptomania | 19 | 928933 | 928952 | 325 | 344 | 16669202 | 1.04 | 0.62 | 1.66 | 0.06 | -0.71 | 0.60 | 1.04 (0.62-1.66) [19] | 0.06 (-0.71-0.6) [19] | FALSE | no SDR | no SDR |
TGAs | kleptomania | 197 | 128309 | 128506 | 147 | 344 | 17469826 | 182.18 | 146.94 | 229.05 | 6.03 | 5.79 | 6.20 | 182.18 (146.94-229.05) [197] | 6.03 (5.79-6.2) [197] | TRUE | SDR | SDR |
aripiprazole | kleptomania | 195 | 111352 | 111547 | 149 | 344 | 17486783 | 206.74 | 164.42 | 258.48 | 6.18 | 5.95 | 6.36 | 206.74 (164.42-258.48) [195] | 6.18 (5.95-6.36) [195] | TRUE | SDR | SDR |
pramipexole | kleptomania | 17 | 33545 | 33562 | 327 | 344 | 17564590 | 27.23 | 15.65 | 44.28 | 3.92 | 3.10 | 4.48 | 27.23 (15.65-44.28) [17] | 3.92 (3.1-4.48) [17] | TRUE | SDR | SDR |
paracetamol | stereotypy | 28 | 928924 | 928952 | 974 | 1002 | 16668553 | 0.51 | 0.34 | 0.75 | -0.91 | -1.54 | -0.46 | 0.51 (0.34-0.75) [28] | -0.91 (-1.54–0.46) [28] | FALSE | no SDR | no SDR |
TGAs | stereotypy | 63 | 128443 | 128506 | 939 | 1002 | 17469034 | 9.12 | 6.95 | 11.78 | 3.02 | 2.60 | 3.32 | 9.12 (6.95-11.78) [63] | 3.02 (2.6-3.32) [63] | TRUE | SDR | SDR |
aripiprazole | stereotypy | 60 | 111487 | 111547 | 942 | 1002 | 17485990 | 9.98 | 7.56 | 12.97 | 3.14 | 2.71 | 3.45 | 9.98 (7.56-12.97) [60] | 3.14 (2.71-3.45) [60] | TRUE | SDR | SDR |
pramipexole | stereotypy | 96 | 33466 | 33562 | 906 | 1002 | 17564011 | 55.63 | 44.52 | 68.79 | 5.32 | 4.98 | 5.56 | 55.63 (44.52-68.79) [96] | 5.32 (4.98-5.56) [96] | TRUE | SDR | SDR |
paracetamol | excessive exercise | 5 | 928947 | 928952 | 80 | 85 | 16669447 | 1.12 | 0.35 | 2.72 | 0.14 | -1.43 | 1.12 | 1.12 (0.35-2.72) [5] | 0.14 (-1.43-1.12) [5] | FALSE | no SDR | no SDR |
TGAs | excessive exercise | 2 | 128504 | 128506 | 83 | 85 | 17469890 | 3.27 | 0.39 | 12.21 | 1.15 | -1.44 | 2.54 | 3.27 (0.39-12.21) [2] | 1.15 (-1.44-2.54) [2] | FALSE | not enough cases | not enough cases |
aripiprazole | excessive exercise | 1 | 111546 | 111547 | 84 | 85 | 17486848 | 1.86 | 0.04 | 10.68 | 0.53 | -3.26 | 2.21 | 1.86 (0.04-10.68) [1] | 0.53 (-3.26-2.21) [1] | FALSE | not enough cases | not enough cases |
pramipexole | excessive exercise | 7 | 33555 | 33562 | 78 | 85 | 17564839 | 46.93 | 18.28 | 101.36 | 3.50 | 2.19 | 4.35 | 46.93 (18.28-101.36) [7] | 3.5 (2.19-4.35) [7] | TRUE | SDR | SDR |
paracetamol | pyromania | 1 | 928951 | 928952 | 96 | 97 | 16669431 | 0.18 | 0.00 | 1.06 | -1.91 | -5.69 | -0.22 | 0.18 (0-1.06) [1] | -1.91 (-5.69–0.22) [1] | FALSE | not enough cases | not enough cases |
TGAs | pyromania | 6 | 128500 | 128506 | 91 | 97 | 17469882 | 8.96 | 3.20 | 20.27 | 2.42 | 1.01 | 3.33 | 8.96 (3.2-20.27) [6] | 2.42 (1.01-3.33) [6] | TRUE | SDR | SDR |
aripiprazole | pyromania | 6 | 111541 | 111547 | 91 | 97 | 17486841 | 10.33 | 3.69 | 23.36 | 2.54 | 1.12 | 3.45 | 10.33 (3.69-23.36) [6] | 2.54 (1.12-3.45) [6] | TRUE | SDR | SDR |
pramipexole | pyromania | 1 | 33561 | 33562 | 96 | 97 | 17564821 | 5.45 | 0.13 | 31.11 | 1.13 | -2.66 | 2.81 | 5.45 (0.13-31.11) [1] | 1.13 (-2.66-2.81) [1] | FALSE | not enough cases | not enough cases |
paracetamol | poriomania | 6 | 928946 | 928952 | 167 | 173 | 16669360 | 0.64 | 0.23 | 1.43 | -0.57 | -1.99 | 0.34 | 0.64 (0.23-1.43) [6] | -0.57 (-1.99-0.34) [6] | FALSE | no SDR | no SDR |
TGAs | poriomania | 16 | 128490 | 128506 | 157 | 173 | 17469816 | 13.86 | 7.72 | 23.21 | 3.22 | 2.38 | 3.80 | 13.86 (7.72-23.21) [16] | 3.22 (2.38-3.8) [16] | TRUE | SDR | SDR |
aripiprazole | poriomania | 16 | 111531 | 111547 | 157 | 173 | 17486775 | 15.97 | 8.91 | 26.77 | 3.36 | 2.52 | 3.95 | 15.97 (8.91-26.77) [16] | 3.36 (2.52-3.95) [16] | TRUE | SDR | SDR |
pramipexole | poriomania | 2 | 33560 | 33562 | 171 | 173 | 17564746 | 6.12 | 0.73 | 22.45 | 1.59 | -1.01 | 2.98 | 6.12 (0.73-22.45) [2] | 1.59 (-1.01-2.98) [2] | FALSE | not enough cases | not enough cases |
paracetamol | overwork | 13 | 928939 | 928952 | 59 | 72 | 16669468 | 3.95 | 1.98 | 7.29 | 1.65 | 0.71 | 2.29 | 3.95 (1.98-7.29) [13] | 1.65 (0.71-2.29) [13] | TRUE | SDR | SDR |
TGAs | overwork | 2 | 128504 | 128506 | 70 | 72 | 17469903 | 3.88 | 0.46 | 14.56 | 1.28 | -1.31 | 2.67 | 3.88 (0.46-14.56) [2] | 1.28 (-1.31-2.67) [2] | FALSE | not enough cases | not enough cases |
aripiprazole | overwork | 2 | 111545 | 111547 | 70 | 72 | 17486862 | 4.47 | 0.53 | 16.79 | 1.38 | -1.21 | 2.77 | 4.47 (0.53-16.79) [2] | 1.38 (-1.21-2.77) [2] | FALSE | not enough cases | not enough cases |
pramipexole | overwork | 0 | 33562 | 33562 | 72 | 72 | 17564845 | 0.00 | 0.00 | 27.53 | -0.36 | -10.68 | 1.62 | 0 (0-27.53) [0] | -0.36 (-10.68-1.62) [0] | FALSE | not enough cases | not enough cases |
paracetamol | liver injury | 3417 | 925535 | 928952 | 13385 | 16802 | 16656142 | 4.59 | 4.42 | 4.77 | 1.94 | 1.88 | 1.98 | 4.59 (4.42-4.77) [3417] | 1.94 (1.88-1.98) [3417] | TRUE | SDR | SDR |
TGAs | liver injury | 122 | 128384 | 128506 | 16680 | 16802 | 17453293 | 0.99 | 0.82 | 1.18 | -0.01 | -0.31 | 0.20 | 0.99 (0.82-1.18) [122] | -0.01 (-0.31-0.2) [122] | FALSE | no SDR | no SDR |
aripiprazole | liver injury | 120 | 111427 | 111547 | 16682 | 16802 | 17470250 | 1.12 | 0.93 | 1.34 | 0.17 | -0.14 | 0.38 | 1.12 (0.93-1.34) [120] | 0.17 (-0.14-0.38) [120] | FALSE | no SDR | no SDR |
pramipexole | liver injury | 20 | 33542 | 33562 | 16782 | 16802 | 17548135 | 0.62 | 0.38 | 0.96 | -0.67 | -1.42 | -0.15 | 0.62 (0.38-0.96) [20] | -0.67 (-1.42–0.15) [20] | FALSE | no SDR | no SDR |
paracetamol | compulsive hoarding | 23 | 928929 | 928952 | 297 | 320 | 16669230 | 1.38 | 0.86 | 2.12 | 0.43 | -0.27 | 0.92 | 1.38 (0.86-2.12) [23] | 0.43 (-0.27-0.92) [23] | FALSE | no SDR | no SDR |
TGAs | compulsive hoarding | 247 | 128259 | 128506 | 73 | 320 | 17469900 | 456.46 | 353.86 | 601.67 | 6.44 | 6.23 | 6.59 | 456.46 (353.86-601.67) [247] | 6.44 (6.23-6.59) [247] | TRUE | SDR | SDR |
aripiprazole | compulsive hoarding | 245 | 111302 | 111547 | 75 | 320 | 17486857 | 511.88 | 396.39 | 674.49 | 6.60 | 6.39 | 6.75 | 511.88 (396.39-674.49) [245] | 6.6 (6.39-6.75) [245] | TRUE | SDR | SDR |
pramipexole | compulsive hoarding | 29 | 33533 | 33562 | 291 | 320 | 17564626 | 52.23 | 34.34 | 76.52 | 4.73 | 4.11 | 5.17 | 52.23 (34.34-76.52) [29] | 4.73 (4.11-5.17) [29] | TRUE | SDR | SDR |
paracetamol | compulsive shopping | 117 | 928835 | 928952 | 1773 | 1890 | 16667754 | 1.18 | 0.97 | 1.42 | 0.22 | -0.08 | 0.44 | 1.18 (0.97-1.42) [117] | 0.22 (-0.08-0.44) [117] | FALSE | no SDR | no SDR |
TGAs | compulsive shopping | 1147 | 127359 | 128506 | 743 | 1890 | 17469230 | 211.44 | 193.40 | 232.40 | 6.32 | 6.22 | 6.39 | 211.44 (193.4-232.4) [1147] | 6.32 (6.22-6.39) [1147] | TRUE | SDR | SDR |
aripiprazole | compulsive shopping | 1108 | 110439 | 111547 | 782 | 1890 | 17486150 | 224.01 | 203.60 | 247.78 | 6.47 | 6.37 | 6.54 | 224.01 (203.6-247.78) [1108] | 6.47 (6.37-6.54) [1108] | TRUE | SDR | SDR |
pramipexole | compulsive shopping | 412 | 33150 | 33562 | 1478 | 1890 | 17563439 | 147.02 | 132.34 | 164.62 | 6.65 | 6.48 | 6.76 | 147.02 (132.34-164.62) [412] | 6.65 (6.48-6.76) [412] | TRUE | SDR | SDR |
paracetamol | gaming disorder | 0 | 928952 | 928952 | 9 | 9 | 16669518 | 0.00 | 0.00 | 9.09 | -0.97 | -11.29 | 1.01 | 0 (0-9.09) [0] | -0.97 (-11.29-1.01) [0] | FALSE | not enough cases | not enough cases |
TGAs | gaming disorder | 3 | 128503 | 128506 | 6 | 9 | 17469967 | 67.95 | 10.99 | 317.97 | 2.62 | 0.55 | 3.83 | 67.95 (10.99-317.97) [3] | 2.62 (0.55-3.83) [3] | TRUE | SDR | SDR |
aripiprazole | gaming disorder | 3 | 111544 | 111547 | 6 | 9 | 17486926 | 78.38 | 12.68 | 365.96 | 2.65 | 0.58 | 3.85 | 78.38 (12.68-365.96) [3] | 2.65 (0.58-3.85) [3] | TRUE | SDR | SDR |
pramipexole | gaming disorder | 3 | 33559 | 33562 | 6 | 9 | 17564911 | 262.52 | 42.34 | 1215.32 | 2.75 | 0.68 | 3.96 | 262.52 (42.34-1215.32) [3] | 2.75 (0.68-3.96) [3] | TRUE | SDR | SDR |
render_forest(disproportionality_df, row = "event", facet_v = "substance")
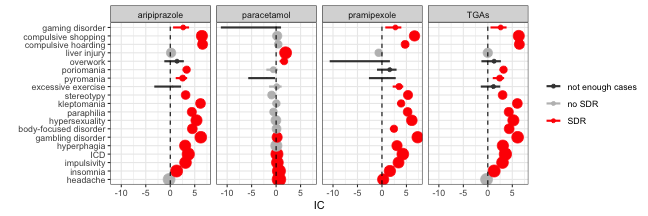
Here we see an extensive association of impulsive manifestations with aripiprazole, its class (which is in fact almost the same as aripiprazole as the other two drugs are far less used), and the positive control pramipexole (which shows also enough cases with excessive exercise, even if not enough cases with pyromania). Aripiprazole is not associated with liver injury and headache (as expected), while instead paracetamol is associated with both, the first a known reaction, the second a reverse causality (you take paracetamol to treat headache). Paracetamol is not associated with many of the impulsivity manifestations, but it is still associated with impulsivity, ICD, gambling and overwork (you see the red dots that in this plot identify an SDR). Some possible explanations may be present: overwork and the use of paracetamol to fight the subsequent migraine have a comprehensible association, but also impulsivity intended as a more general irritability due to the pain.
Focusing on suspected drugs
In fact, paracetamol is used so much that it could be in many cases just recorded as a concomitant. For this reason the next step is performing the same analysis only considering suspected drugs. We are not going to change anymore the definition section. Let’s work only on the disproportionality one, specifying in the temp_drug (the drug database we want to use, which is the entire drug by default) only drugs which are recorded as primary or secondary suspect (rol_cod %in% c(“PS”,“SS”)). Remember to respond “no” in the console when the function asks you if you want to refine the query.
## Disproportionality analysis ----------------------------------------------------------
# Suspected drugs
disproportionality_df <- disproportionality_analysis(
drug_selected = drug_selected,
reac_selected = reac_selected,
temp_drug = Drug[role_cod %in% c("PS", "SS")]
)
## Definitions --------------------------------------------------------------------------
disproportionality_df
substance | event | D_E | D_nE | D | nD_E | E | nD_nE | ROR_median | ROR_lower | ROR_upper | IC_median | IC_lower | IC_upper | label_ROR | label_IC | Bonferroni | ROR_signal | IC_signal |
---|---|---|---|---|---|---|---|---|---|---|---|---|---|---|---|---|---|---|
paracetamol | headache | 8275 | 334670 | 342945 | 529350 | 537625 | 16725628 | 0.78 | 0.76 | 0.79 | -0.35 | -0.38 | -0.32 | 0.78 (0.76-0.79) [8275] | -0.35 (-0.38–0.32) [8275] | FALSE | no SDR | no SDR |
aripiprazole | headache | 1697 | 79728 | 81425 | 535928 | 537625 | 16980570 | 0.67 | 0.64 | 0.70 | -0.56 | -0.64 | -0.50 | 0.67 (0.64-0.7) [1697] | -0.56 (-0.64–0.5) [1697] | FALSE | no SDR | no SDR |
TGAs | headache | 1970 | 94009 | 95979 | 535655 | 537625 | 16966289 | 0.66 | 0.63 | 0.69 | -0.58 | -0.65 | -0.52 | 0.66 (0.63-0.69) [1970] | -0.58 (-0.65–0.52) [1970] | FALSE | no SDR | no SDR |
pramipexole | headache | 269 | 10828 | 11097 | 537356 | 537625 | 17049470 | 0.78 | 0.69 | 0.88 | -0.34 | -0.54 | -0.19 | 0.78 (0.69-0.88) [269] | -0.34 (-0.54–0.19) [269] | FALSE | no SDR | no SDR |
paracetamol | insomnia | 4630 | 338315 | 342945 | 227624 | 232254 | 17027354 | 1.02 | 0.99 | 1.05 | 0.03 | -0.02 | 0.06 | 1.02 (0.99-1.05) [4630] | 0.03 (-0.02-0.06) [4630] | FALSE | no SDR | no SDR |
aripiprazole | insomnia | 2749 | 78676 | 81425 | 229505 | 232254 | 17286993 | 2.63 | 2.53 | 2.73 | 1.35 | 1.29 | 1.40 | 2.63 (2.53-2.73) [2749] | 1.35 (1.29-1.4) [2749] | TRUE | weak SDR | SDR |
TGAs | insomnia | 3192 | 92787 | 95979 | 229062 | 232254 | 17272882 | 2.59 | 2.50 | 2.68 | 1.33 | 1.27 | 1.37 | 2.59 (2.5-2.68) [3192] | 1.33 (1.27-1.37) [3192] | TRUE | weak SDR | SDR |
pramipexole | insomnia | 554 | 10543 | 11097 | 231700 | 232254 | 17355126 | 3.93 | 3.60 | 4.28 | 1.91 | 1.77 | 2.01 | 3.93 (3.6-4.28) [554] | 1.91 (1.77-2.01) [554] | TRUE | weak SDR | SDR |
paracetamol | impulsivity | 791 | 342154 | 342945 | 41297 | 42088 | 17213681 | 0.96 | 0.89 | 1.03 | -0.06 | -0.17 | 0.03 | 0.96 (0.89-1.03) [791] | -0.06 (-0.17-0.03) [791] | FALSE | no SDR | no SDR |
aripiprazole | impulsivity | 1901 | 79524 | 81425 | 40187 | 42088 | 17476311 | 10.39 | 9.91 | 10.89 | 3.28 | 3.20 | 3.33 | 10.39 (9.91-10.89) [1901] | 3.28 (3.2-3.33) [1901] | TRUE | weak SDR | SDR |
TGAs | impulsivity | 2029 | 93950 | 95979 | 40059 | 42088 | 17461885 | 9.41 | 8.99 | 9.84 | 3.14 | 3.06 | 3.19 | 9.41 (8.99-9.84) [2029] | 3.14 (3.06-3.19) [2029] | TRUE | weak SDR | SDR |
pramipexole | impulsivity | 667 | 10430 | 11097 | 41421 | 42088 | 17545405 | 27.09 | 25.00 | 29.32 | 4.62 | 4.49 | 4.71 | 27.09 (25-29.32) [667] | 4.62 (4.49-4.71) [667] | TRUE | weak SDR | SDR |
paracetamol | ICD | 962 | 341983 | 342945 | 71334 | 72296 | 17183644 | 0.67 | 0.63 | 0.72 | -0.56 | -0.66 | -0.48 | 0.67 (0.63-0.72) [962] | -0.56 (-0.66–0.48) [962] | FALSE | no SDR | no SDR |
aripiprazole | ICD | 5208 | 76217 | 81425 | 67088 | 72296 | 17449410 | 17.77 | 17.25 | 18.30 | 3.95 | 3.91 | 3.99 | 17.77 (17.25-18.3) [5208] | 3.95 (3.91-3.99) [5208] | TRUE | weak SDR | SDR |
TGAs | ICD | 5666 | 90313 | 95979 | 66630 | 72296 | 17435314 | 16.41 | 15.96 | 16.89 | 3.84 | 3.79 | 3.87 | 16.41 (15.96-16.89) [5666] | 3.84 (3.79-3.87) [5666] | TRUE | weak SDR | SDR |
pramipexole | ICD | 2376 | 8721 | 11097 | 69920 | 72296 | 17516906 | 68.26 | 65.14 | 71.25 | 5.68 | 5.62 | 5.73 | 68.26 (65.14-71.25) [2376] | 5.68 (5.62-5.73) [2376] | TRUE | weak SDR | SDR |
paracetamol | hyperphagia | 108 | 342837 | 342945 | 21166 | 21274 | 17233812 | 0.25 | 0.21 | 0.30 | -1.94 | -2.26 | -1.71 | 0.25 (0.21-0.3) [108] | -1.94 (-2.26–1.71) [108] | FALSE | no SDR | no SDR |
aripiprazole | hyperphagia | 935 | 80490 | 81425 | 20339 | 21274 | 17496159 | 9.99 | 9.34 | 10.67 | 3.24 | 3.13 | 3.31 | 9.99 (9.34-10.67) [935] | 3.24 (3.13-3.31) [935] | TRUE | weak SDR | SDR |
TGAs | hyperphagia | 1124 | 94855 | 95979 | 20150 | 21274 | 17481794 | 10.27 | 9.67 | 10.92 | 3.27 | 3.17 | 3.34 | 10.27 (9.67-10.92) [1124] | 3.27 (3.17-3.34) [1124] | TRUE | weak SDR | SDR |
pramipexole | hyperphagia | 293 | 10804 | 11097 | 20981 | 21274 | 17565845 | 22.70 | 20.12 | 25.51 | 4.39 | 4.20 | 4.53 | 22.7 (20.12-25.51) [293] | 4.39 (4.2-4.53) [293] | TRUE | weak SDR | SDR |
paracetamol | gambling disorder | 9 | 342936 | 342945 | 5059 | 5068 | 17249919 | 0.08 | 0.04 | 0.17 | -3.39 | -4.53 | -2.63 | 0.08 (0.04-0.17) [9] | -3.39 (-4.53–2.63) [9] | FALSE | no SDR | no SDR |
aripiprazole | gambling disorder | 2470 | 78955 | 81425 | 2598 | 5068 | 17513900 | 210.01 | 199.18 | 223.51 | 6.68 | 6.62 | 6.73 | 210.01 (199.18-223.51) [2470] | 6.68 (6.62-6.73) [2470] | TRUE | weak SDR | SDR |
TGAs | gambling disorder | 2559 | 93420 | 95979 | 2509 | 5068 | 17499435 | 192.03 | 180.40 | 202.70 | 6.50 | 6.44 | 6.55 | 192.03 (180.4-202.7) [2559] | 6.5 (6.44-6.55) [2559] | TRUE | weak SDR | SDR |
pramipexole | gambling disorder | 1543 | 9554 | 11097 | 3525 | 5068 | 17583301 | 803.07 | 748.12 | 869.09 | 8.70 | 8.62 | 8.76 | 803.07 (748.12-869.09) [1543] | 8.7 (8.62-8.76) [1543] | TRUE | weak SDR | SDR |
paracetamol | body-focused disorder | 18 | 342927 | 342945 | 1291 | 1309 | 17253687 | 0.70 | 0.41 | 1.11 | -0.50 | -1.29 | 0.06 | 0.7 (0.41-1.11) [18] | -0.5 (-1.29-0.06) [18] | FALSE | no SDR | no SDR |
aripiprazole | body-focused disorder | 190 | 81235 | 81425 | 1119 | 1309 | 17515379 | 36.60 | 31.21 | 42.79 | 4.86 | 4.62 | 5.03 | 36.6 (31.21-42.79) [190] | 4.86 (4.62-5.03) [190] | TRUE | weak SDR | SDR |
TGAs | body-focused disorder | 194 | 95785 | 95979 | 1115 | 1309 | 17500829 | 31.79 | 27.12 | 37.07 | 4.67 | 4.43 | 4.84 | 31.79 (27.12-37.07) [194] | 4.67 (4.43-4.84) [194] | TRUE | weak SDR | SDR |
pramipexole | body-focused disorder | 13 | 11084 | 11097 | 1296 | 1309 | 17585530 | 15.91 | 8.44 | 27.32 | 3.34 | 2.40 | 3.99 | 15.91 (8.44-27.32) [13] | 3.34 (2.4-3.99) [13] | TRUE | weak SDR | SDR |
paracetamol | hypersexuality | 10 | 342935 | 342945 | 4186 | 4196 | 17250792 | 0.12 | 0.05 | 0.22 | -2.97 | -4.05 | -2.25 | 0.12 (0.05-0.22) [10] | -2.97 (-4.05–2.25) [10] | FALSE | no SDR | no SDR |
aripiprazole | hypersexuality | 1050 | 80375 | 81425 | 3146 | 4196 | 17513352 | 72.72 | 67.81 | 78.04 | 5.72 | 5.61 | 5.79 | 72.72 (67.81-78.04) [1050] | 5.72 (5.61-5.79) [1050] | TRUE | weak SDR | SDR |
TGAs | hypersexuality | 1092 | 94887 | 95979 | 3104 | 4196 | 17498840 | 64.89 | 60.39 | 69.69 | 5.54 | 5.44 | 5.61 | 64.89 (60.39-69.69) [1092] | 5.54 (5.44-5.61) [1092] | TRUE | weak SDR | SDR |
pramipexole | hypersexuality | 544 | 10553 | 11097 | 3652 | 4196 | 17583174 | 249.04 | 224.63 | 270.52 | 7.43 | 7.29 | 7.53 | 249.04 (224.63-270.52) [544] | 7.43 (7.29-7.53) [544] | TRUE | weak SDR | SDR |
paracetamol | paraphilia | 7 | 342938 | 342945 | 596 | 603 | 17254382 | 0.59 | 0.23 | 1.22 | -0.71 | -2.02 | 0.14 | 0.59 (0.23-1.22) [7] | -0.71 (-2.02-0.14) [7] | FALSE | no SDR | no SDR |
aripiprazole | paraphilia | 83 | 81342 | 81425 | 520 | 603 | 17515978 | 34.37 | 26.92 | 43.39 | 4.66 | 4.30 | 4.92 | 34.37 (26.92-43.39) [83] | 4.66 (4.3-4.92) [83] | TRUE | weak SDR | SDR |
TGAs | paraphilia | 85 | 95894 | 95979 | 518 | 603 | 17501426 | 29.95 | 23.51 | 37.70 | 4.49 | 4.13 | 4.75 | 29.95 (23.51-37.7) [85] | 4.49 (4.13-4.75) [85] | TRUE | weak SDR | SDR |
pramipexole | paraphilia | 61 | 11036 | 11097 | 542 | 603 | 17586284 | 180.22 | 135.62 | 232.69 | 6.12 | 5.70 | 6.43 | 180.22 (135.62-232.69) [61] | 6.12 (5.7-6.43) [61] | TRUE | weak SDR | SDR |
paracetamol | kleptomania | 3 | 342942 | 342945 | 341 | 344 | 17254637 | 0.44 | 0.09 | 1.30 | -1.05 | -3.12 | 0.16 | 0.44 (0.09-1.3) [3] | -1.05 (-3.12-0.16) [3] | FALSE | no SDR | no SDR |
aripiprazole | kleptomania | 193 | 81232 | 81425 | 151 | 344 | 17516347 | 274.75 | 220.79 | 349.42 | 6.53 | 6.29 | 6.70 | 274.75 (220.79-349.42) [193] | 6.53 (6.29-6.7) [193] | TRUE | weak SDR | SDR |
TGAs | kleptomania | 195 | 95784 | 95979 | 149 | 344 | 17501795 | 240.10 | 192.47 | 297.18 | 6.36 | 6.12 | 6.53 | 240.1 (192.47-297.18) [195] | 6.36 (6.12-6.53) [195] | TRUE | weak SDR | SDR |
pramipexole | kleptomania | 13 | 11084 | 11097 | 331 | 344 | 17586495 | 62.35 | 32.82 | 108.31 | 4.23 | 3.29 | 4.87 | 62.35 (32.82-108.31) [13] | 4.23 (3.29-4.87) [13] | TRUE | weak SDR | SDR |
paracetamol | stereotypy | 11 | 342934 | 342945 | 991 | 1002 | 17253987 | 0.55 | 0.27 | 1.00 | -0.81 | -1.83 | -0.11 | 0.55 (0.27-1) [11] | -0.81 (-1.83–0.11) [11] | FALSE | no SDR | no SDR |
aripiprazole | stereotypy | 55 | 81370 | 81425 | 947 | 1002 | 17515551 | 12.50 | 9.34 | 16.41 | 3.43 | 2.98 | 3.75 | 12.5 (9.34-16.41) [55] | 3.43 (2.98-3.75) [55] | TRUE | weak SDR | SDR |
TGAs | stereotypy | 58 | 95921 | 95979 | 944 | 1002 | 17501000 | 11.21 | 8.44 | 14.62 | 3.29 | 2.85 | 3.60 | 11.21 (8.44-14.62) [58] | 3.29 (2.85-3.6) [58] | TRUE | weak SDR | SDR |
pramipexole | stereotypy | 87 | 11010 | 11097 | 915 | 1002 | 17585911 | 152.33 | 120.89 | 188.92 | 6.27 | 5.91 | 6.52 | 152.33 (120.89-188.92) [87] | 6.27 (5.91-6.52) [87] | TRUE | weak SDR | SDR |
paracetamol | excessive exercise | 1 | 342944 | 342945 | 84 | 85 | 17254894 | 0.59 | 0.01 | 3.42 | -0.53 | -4.31 | 1.16 | 0.59 (0.01-3.42) [1] | -0.53 (-4.31-1.16) [1] | FALSE | not enough cases | not enough cases |
aripiprazole | excessive exercise | 1 | 81424 | 81425 | 84 | 85 | 17516414 | 2.56 | 0.06 | 14.66 | 0.74 | -3.04 | 2.43 | 2.56 (0.06-14.66) [1] | 0.74 (-3.04-2.43) [1] | FALSE | not enough cases | not enough cases |
TGAs | excessive exercise | 2 | 95977 | 95979 | 83 | 85 | 17501861 | 4.39 | 0.52 | 16.37 | 1.37 | -1.22 | 2.76 | 4.39 (0.52-16.37) [2] | 1.37 (-1.22-2.76) [2] | FALSE | not enough cases | not enough cases |
pramipexole | excessive exercise | 7 | 11090 | 11097 | 78 | 85 | 17586748 | 142.26 | 55.35 | 306.66 | 3.75 | 2.45 | 4.61 | 142.26 (55.35-306.66) [7] | 3.75 (2.45-4.61) [7] | TRUE | weak SDR | SDR |
paracetamol | pyromania | 1 | 342944 | 342945 | 96 | 97 | 17254882 | 0.52 | 0.01 | 2.99 | -0.68 | -4.46 | 1.01 | 0.52 (0.01-2.99) [1] | -0.68 (-4.46-1.01) [1] | FALSE | not enough cases | not enough cases |
aripiprazole | pyromania | 6 | 81419 | 81425 | 91 | 97 | 17516407 | 14.18 | 5.07 | 32.10 | 2.77 | 1.36 | 3.68 | 14.18 (5.07-32.1) [6] | 2.77 (1.36-3.68) [6] | TRUE | weak SDR | SDR |
TGAs | pyromania | 6 | 95973 | 95979 | 91 | 97 | 17501853 | 12.02 | 4.29 | 27.19 | 2.65 | 1.24 | 3.57 | 12.02 (4.29-27.19) [6] | 2.65 (1.24-3.57) [6] | TRUE | weak SDR | SDR |
pramipexole | pyromania | 1 | 11096 | 11097 | 96 | 97 | 17586730 | 16.50 | 0.41 | 93.96 | 1.41 | -2.37 | 3.10 | 16.5 (0.41-93.96) [1] | 1.41 (-2.37-3.1) [1] | FALSE | not enough cases | not enough cases |
paracetamol | poriomania | 2 | 342943 | 342945 | 171 | 173 | 17254807 | 0.58 | 0.07 | 2.15 | -0.64 | -3.23 | 0.76 | 0.58 (0.07-2.15) [2] | -0.64 (-3.23-0.76) [2] | FALSE | not enough cases | not enough cases |
aripiprazole | poriomania | 12 | 81413 | 81425 | 161 | 173 | 17516337 | 16.03 | 8.11 | 28.79 | 3.26 | 2.28 | 3.93 | 16.03 (8.11-28.79) [12] | 3.26 (2.28-3.93) [12] | TRUE | weak SDR | SDR |
TGAs | poriomania | 12 | 95967 | 95979 | 161 | 173 | 17501783 | 13.58 | 6.87 | 24.38 | 3.11 | 2.13 | 3.78 | 13.58 (6.87-24.38) [12] | 3.11 (2.13-3.78) [12] | TRUE | weak SDR | SDR |
pramipexole | poriomania | 1 | 11096 | 11097 | 172 | 173 | 17586654 | 9.21 | 0.23 | 52.12 | 1.30 | -2.49 | 2.98 | 9.21 (0.23-52.12) [1] | 1.3 (-2.49-2.98) [1] | FALSE | not enough cases | not enough cases |
paracetamol | overwork | 11 | 342934 | 342945 | 61 | 72 | 17254917 | 9.07 | 4.30 | 17.40 | 2.59 | 1.57 | 3.29 | 9.07 (4.3-17.4) [11] | 2.59 (1.57-3.29) [11] | TRUE | weak SDR | SDR |
aripiprazole | overwork | 2 | 81423 | 81425 | 70 | 72 | 17516428 | 6.14 | 0.72 | 23.04 | 1.58 | -1.01 | 2.97 | 6.14 (0.72-23.04) [2] | 1.58 (-1.01-2.97) [2] | FALSE | not enough cases | not enough cases |
TGAs | overwork | 2 | 95977 | 95979 | 70 | 72 | 17501874 | 5.21 | 0.61 | 19.53 | 1.48 | -1.11 | 2.87 | 5.21 (0.61-19.53) [2] | 1.48 (-1.11-2.87) [2] | FALSE | not enough cases | not enough cases |
pramipexole | overwork | 0 | 11097 | 11097 | 72 | 72 | 17586754 | 0.00 | 0.00 | 83.42 | -0.13 | -10.45 | 1.85 | 0 (0-83.42) [0] | -0.13 (-10.45-1.85) [0] | FALSE | not enough cases | not enough cases |
paracetamol | liver injury | 2704 | 340241 | 342945 | 14098 | 16802 | 17240880 | 9.71 | 9.32 | 10.12 | 3.04 | 2.98 | 3.09 | 9.71 (9.32-10.12) [2704] | 3.04 (2.98-3.09) [2704] | TRUE | weak SDR | SDR |
aripiprazole | liver injury | 75 | 81350 | 81425 | 16727 | 16802 | 17499771 | 0.96 | 0.75 | 1.20 | -0.06 | -0.44 | 0.22 | 0.96 (0.75-1.2) [75] | -0.06 (-0.44-0.22) [75] | FALSE | no SDR | no SDR |
TGAs | liver injury | 76 | 95903 | 95979 | 16726 | 16802 | 17485218 | 0.82 | 0.65 | 1.03 | -0.27 | -0.65 | 0.00 | 0.82 (0.65-1.03) [76] | -0.27 (-0.65-0) [76] | FALSE | no SDR | no SDR |
pramipexole | liver injury | 2 | 11095 | 11097 | 16800 | 16802 | 17570026 | 0.18 | 0.02 | 0.68 | -2.15 | -4.75 | -0.76 | 0.18 (0.02-0.68) [2] | -2.15 (-4.75–0.76) [2] | FALSE | not enough cases | not enough cases |
paracetamol | compulsive hoarding | 0 | 342945 | 342945 | 320 | 320 | 17254658 | 0.00 | 0.00 | 0.58 | -3.76 | -14.08 | -1.78 | 0 (0-0.58) [0] | -3.76 (-14.08–1.78) [0] | FALSE | not enough cases | not enough cases |
aripiprazole | compulsive hoarding | 244 | 81181 | 81425 | 76 | 320 | 17516422 | 695.29 | 525.12 | 931.36 | 6.94 | 6.73 | 7.10 | 695.29 (525.12-931.36) [244] | 6.94 (6.73-7.1) [244] | TRUE | weak SDR | SDR |
TGAs | compulsive hoarding | 246 | 95733 | 95979 | 74 | 320 | 17501870 | 605.63 | 460.86 | 788.10 | 6.77 | 6.56 | 6.93 | 605.63 (460.86-788.1) [246] | 6.77 (6.56-6.93) [246] | TRUE | weak SDR | SDR |
pramipexole | compulsive hoarding | 22 | 11075 | 11097 | 298 | 320 | 17586528 | 117.24 | 72.43 | 181.07 | 5.00 | 4.28 | 5.50 | 117.24 (72.43-181.07) [22] | 5 (4.28-5.5) [22] | TRUE | weak SDR | SDR |
paracetamol | compulsive shopping | 8 | 342937 | 342945 | 1882 | 1890 | 17253096 | 0.21 | 0.09 | 0.42 | -2.14 | -3.35 | -1.34 | 0.21 (0.09-0.42) [8] | -2.14 (-3.35–1.34) [8] | FALSE | no SDR | no SDR |
aripiprazole | compulsive shopping | 1104 | 80321 | 81425 | 786 | 1890 | 17515712 | 309.00 | 280.54 | 339.42 | 6.90 | 6.80 | 6.97 | 309 (280.54-339.42) [1104] | 6.9 (6.8-6.97) [1104] | TRUE | weak SDR | SDR |
TGAs | compulsive shopping | 1142 | 94837 | 95979 | 748 | 1890 | 17501196 | 283.82 | 257.38 | 311.74 | 6.72 | 6.62 | 6.79 | 283.82 (257.38-311.74) [1142] | 6.72 (6.62-6.79) [1142] | TRUE | weak SDR | SDR |
pramipexole | compulsive shopping | 396 | 10701 | 11097 | 1494 | 1890 | 17585332 | 438.15 | 389.86 | 496.30 | 7.87 | 7.70 | 7.99 | 438.15 (389.86-496.3) [396] | 7.87 (7.7-7.99) [396] | TRUE | weak SDR | SDR |
paracetamol | gaming disorder | 0 | 342945 | 342945 | 9 | 9 | 17254969 | 0.00 | 0.00 | 25.49 | -0.44 | -10.76 | 1.54 | 0 (0-25.49) [0] | -0.44 (-10.76-1.54) [0] | FALSE | not enough cases | not enough cases |
aripiprazole | gaming disorder | 1 | 81424 | 81425 | 8 | 9 | 17516490 | 26.90 | 0.60 | 201.38 | 1.46 | -2.32 | 3.15 | 26.9 (0.6-201.38) [1] | 1.46 (-2.32-3.15) [1] | FALSE | not enough cases | not enough cases |
TGAs | gaming disorder | 1 | 95978 | 95979 | 8 | 9 | 17501936 | 22.78 | 0.51 | 170.18 | 1.44 | -2.34 | 3.13 | 22.78 (0.51-170.18) [1] | 1.44 (-2.34-3.13) [1] | FALSE | not enough cases | not enough cases |
pramipexole | gaming disorder | 1 | 11096 | 11097 | 8 | 9 | 17586818 | 197.64 | 4.46 | 1431.76 | 1.56 | -2.22 | 3.25 | 197.64 (4.46-1431.76) [1] | 1.56 (-2.22-3.25) [1] | FALSE | not enough cases | not enough cases |
render_forest(disproportionality_df, row = "event", facet_v = "substance")
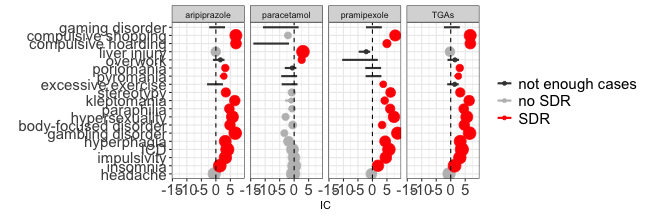
You see this way how all the associations between impulsivity and paracetamol disappear, including, interestingly, also the one with headache (but not the one with overwork).
Removing duplicates
Duplicates may also distort our results. The problem is that we are not sure which reports are duplicates and which are not. Applying a deduplication algorithm may therefore introduce even more distortion. The DiAna package already comes with multiple rule-based strategies to detect potential duplicates, and will soon add even more advanced probabilistic algorithms. The results of these duplicates detection is stored in the DEMO dataset. We therefore have to first import it, going back into the dedicated section and adding the following row together with the other two importing Drug and Reac:
## Import data ----------------------------------------------------------------
import("DEMO")
#> primaryid sex age_in_days wt_in_kgs occr_country event_dt
#> <num> <fctr> <num> <num> <fctr> <int>
#> 1: 45217461 M 9855 NA <NA> 19861106
#> 2: 57910401 <NA> NA NA <NA> NA
#> 3: 56962401 <NA> NA NA <NA> 19960123
#> 4: 54662121 F 22630 NA <NA> 19960919
#> 5: 31231172 F 21535 68 <NA> 19970927
#> ---
#> 17598475: 236937711 F 9490 68 <NA> 20240318
#> 17598476: 236937721 M 6205 NA <NA> 20240101
#> 17598477: 236937741 F 13140 134 <NA> 20240322
#> 17598478: 237079261 F 15695 90 <NA> NA
#> 17598479: 237079291 M 22265 108 <NA> 20240228
#> occp_cod reporter_country rept_cod init_fda_dt fda_dt
#> <fctr> <fctr> <fctr> <int> <int>
#> 1: MD United States of America DIR 19861224 19861224
#> 2: <NA> United States of America DIR 19920917 19920917
#> 3: <NA> United States of America DIR 19960126 19960126
#> 4: CN South Africa, Republic of EXP 19961022 19961022
#> 5: MD United States of America EXP 19971203 19971212
#> ---
#> 17598475: CN United States of America DIR 20240331 20240331
#> 17598476: CN United States of America DIR 20240331 20240331
#> 17598477: CN United States of America DIR 20240331 20240331
#> 17598478: CN United States of America DIR 20240331 20240331
#> 17598479: CN United States of America DIR 20240331 20240331
#> premarketing literature RB_duplicates RB_duplicates_only_susp
#> <lgcl> <lgcl> <lgcl> <lgcl>
#> 1: FALSE FALSE FALSE FALSE
#> 2: FALSE FALSE FALSE FALSE
#> 3: FALSE FALSE FALSE FALSE
#> 4: FALSE FALSE FALSE FALSE
#> 5: FALSE FALSE FALSE FALSE
#> ---
#> 17598475: FALSE FALSE FALSE FALSE
#> 17598476: FALSE FALSE FALSE FALSE
#> 17598477: FALSE FALSE FALSE FALSE
#> 17598478: FALSE FALSE FALSE FALSE
#> 17598479: FALSE FALSE FALSE FALSE
In the printed dataset we see that the last two columns are RB_duplicates and RB_duplicates_only_susp: the first is more conservative, considering as duplicates all the reports that have not only the same sex, age, country, event list, event date, and suspected drug list, but also the concomitants. The second algorithm considers instead only suspected drugs in the drug list.
Let’s reduce the queries considered among the reactions to visualize better the differences in the disproportionality results obtained with the two different algorithms compared to the raw analysis. You can comment the previous generation to reac_selected with a # if you want to keep it and then go back to the previous definition later.
In the disproportionality_analysis section we know include a loop over several possible restrictions of the background reference group: our raw and deduplicated datasets using the different algorithms (raw is the entire dataset, RB1 is the more conservative and RB2 the more speculative deduplication). You will have to answer no in the console every time you are asked (three times).
The disproportionality_df has now a row for each drug-event-background combination, and the background is specified in the “nested” column, which we specified as the “nested” parameter of the render_forest.
## Definitions --------------------------------------------------------------------------
# .....all the content before....
# reac_selected <- rlist::list.append(ICDs,"ICD"=as.list(unlist(purrr::flatten(ICDs))))
reac_selected <- list("ICD" = as.list(unlist(purrr::flatten(ICDs))))
## Disproportionality analysis ----------------------------------------------------------
# Deduplication
restrictions <- list(
"raw" = list(Demo$primaryid),
"RB1" = list(Demo[RB_duplicates == FALSE]$primaryid),
"RB2" = list(Demo[RB_duplicates_only_susp == FALSE]$primaryid)
)
disproportionality_df <- data.table()
for (n in 1:length(restrictions)) {
t <- restrictions[n]
t_name <- names(t)
t_pids <- unlist(t)
df <- disproportionality_analysis(
drug_selected = drug_selected,
reac_selected = reac_selected,
temp_drug = Drug[role_cod %in% c("PS", "SS")],
restriction = t_pids
)[, nested := t_name]
disproportionality_df <- rbindlist(list(disproportionality_df, df), fill = TRUE)
}
## Definitions --------------------------------------------------------------------------
disproportionality_df
substance | event | D_E | D_nE | D | nD_E | E | nD_nE | ROR_median | ROR_lower | ROR_upper | IC_median | IC_lower | IC_upper | label_ROR | label_IC | Bonferroni | ROR_signal | IC_signal | nested |
---|---|---|---|---|---|---|---|---|---|---|---|---|---|---|---|---|---|---|---|
paracetamol | ICD | 962 | 341983 | 342945 | 71334 | 72296 | 17183644 | 0.67 | 0.63 | 0.72 | -0.56 | -0.66 | -0.48 | 0.67 (0.63-0.72) [962] | -0.56 (-0.66–0.48) [962] | FALSE | no SDR | no SDR | raw |
aripiprazole | ICD | 5208 | 76217 | 81425 | 67088 | 72296 | 17449410 | 17.77 | 17.25 | 18.30 | 3.95 | 3.91 | 3.99 | 17.77 (17.25-18.3) [5208] | 3.95 (3.91-3.99) [5208] | TRUE | weak SDR | SDR | raw |
TGAs | ICD | 5666 | 90313 | 95979 | 66630 | 72296 | 17435314 | 16.41 | 15.96 | 16.89 | 3.84 | 3.79 | 3.87 | 16.41 (15.96-16.89) [5666] | 3.84 (3.79-3.87) [5666] | TRUE | weak SDR | SDR | raw |
pramipexole | ICD | 2376 | 8721 | 11097 | 69920 | 72296 | 17516906 | 68.26 | 65.14 | 71.25 | 5.68 | 5.62 | 5.73 | 68.26 (65.14-71.25) [2376] | 5.68 (5.62-5.73) [2376] | TRUE | weak SDR | SDR | raw |
paracetamol | ICD | 930 | 225399 | 226329 | 67187 | 68117 | 14166693 | 0.86 | 0.81 | 0.92 | -0.20 | -0.31 | -0.12 | 0.86 (0.81-0.92) [930] | -0.2 (-0.31–0.12) [930] | FALSE | no SDR | no SDR | RB1 |
aripiprazole | ICD | 4684 | 61702 | 66386 | 63433 | 68117 | 14330390 | 17.16 | 16.62 | 17.69 | 3.90 | 3.85 | 3.93 | 17.16 (16.62-17.69) [4684] | 3.9 (3.85-3.93) [4684] | TRUE | weak SDR | SDR | RB1 |
TGAs | ICD | 5127 | 73112 | 78239 | 62990 | 68117 | 14318980 | 15.94 | 15.48 | 16.41 | 3.79 | 3.75 | 3.82 | 15.94 (15.48-16.41) [5127] | 3.79 (3.75-3.82) [5127] | TRUE | weak SDR | SDR | RB1 |
pramipexole | ICD | 2091 | 7702 | 9793 | 66026 | 68117 | 14384390 | 59.11 | 56.24 | 62.03 | 5.48 | 5.41 | 5.53 | 59.11 (56.24-62.03) [2091] | 5.48 (5.41-5.53) [2091] | TRUE | weak SDR | SDR | RB1 |
paracetamol | ICD | 909 | 210723 | 211632 | 66283 | 67192 | 13701560 | 0.89 | 0.83 | 0.95 | -0.17 | -0.28 | -0.09 | 0.89 (0.83-0.95) [909] | -0.17 (-0.28–0.09) [909] | FALSE | no SDR | no SDR | RB2 |
aripiprazole | ICD | 4530 | 57485 | 62015 | 62662 | 67192 | 13854798 | 17.42 | 16.87 | 17.98 | 3.92 | 3.87 | 3.95 | 17.42 (16.87-17.98) [4530] | 3.92 (3.87-3.95) [4530] | TRUE | weak SDR | SDR | RB2 |
TGAs | ICD | 4958 | 68306 | 73264 | 62234 | 67192 | 13843977 | 16.14 | 15.67 | 16.63 | 3.81 | 3.76 | 3.84 | 16.14 (15.67-16.63) [4958] | 3.81 (3.76-3.84) [4958] | TRUE | weak SDR | SDR | RB2 |
pramipexole | ICD | 2050 | 7260 | 9310 | 65142 | 67192 | 13905023 | 60.29 | 57.27 | 63.24 | 5.50 | 5.42 | 5.55 | 60.29 (57.27-63.24) [2050] | 5.5 (5.42-5.55) [2050] | TRUE | weak SDR | SDR | RB2 |
render_forest(disproportionality_df, row = "event", facet_v = "substance", nested = "nested")
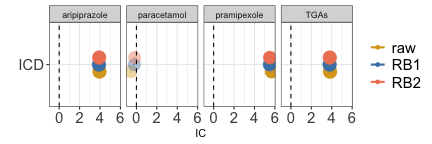
The forest plot shows in fact almost no difference when using the raw analysis compared to the two different rule-based deduplication algorithms available in DiAna for the investigated drug-event combination, resulting in a significant difference only for paracetamol (a signal for impulsivity appears when deduplicating, independently of the algorithm). For most drug-event combinations, we expect to find small differences when deduplicating, but it is always recommended to provide both raw and deduplicated analyses.
Restricting on indication
Another important application made possible by the disproportionality_analysis function is related with addressing expected biases. When investigating a potential causal role of aripiprazole in inducing impulsivity, we should consider that bipolar disorder, a major indication for using aripiprazole, is also a known risk factor for impulsivity and impulsive behaviors.
Could that be sufficient to explain the association (SDR) we found? To address, at least partly, this indication bias and answer this question we can restrict the analysis to patients sharing a diagnosis of bipolar disorder, and checking in this population with homogeneous values for the confounder whether impulsivity is more often recorded together with aripiprazole than with other drugs. For a pedagogical article on how to chose the background reference group (and why restricting on the confounder should be preferred to adjusting on it) see https://doi.org/10.31219/osf.io/h5w9u. We check for the presence of the confounder among the indications, and we therefore have to import the Indi database adding it to the import data section.
## Import data ----------------------------------------------------------------
import("INDI")
#> primaryid drug_seq indi_pt
#> <num> <int> <ord>
#> 1: 4204616 1004278786 abortion induced
#> 2: 4223542 1004334703 crohn's disease
#> 3: 4250482 1004434717 crohn's disease
#> 4: 4261823 1004486217 bronchial carcinoma
#> 5: 4261823 1004486218 bronchial carcinoma
#> ---
#> 41380269: 992001227 583 product used for unknown indication
#> 41380270: 992001227 586 product used for unknown indication
#> 41380271: 236936391 1 beta haemolytic streptococcal infection
#> 41380272: 237079291 1 acrochordon excision
#> 41380273: 237203351 1 product used for unknown indication
We can then search in the Indi dataset for the recording of bipolar disorder, and select their primaryids. To be more exhaustive in the identification of reports concerning patients diagnosed with bipolar disorder, thus collecting greater number of reports for the analysis, we search for bipolar disorder also in the Reac database (confusion may have arisen in the compilation), and for lithium (a specific tratment for bipolar disorder) among the drugs.
## Reference groups --------------------------------------------------------------------
bipolar_disorder_indi_pt <- unique(Indi[indi_pt == "bipolar disorder"]$primaryid)
bipolar_disorder_reac_pt <- unique(Reac[pt == "bipolar disorder"]$primaryid)
bipolar_disorder_drug <- unique(Drug[substance == "lithium"]$primaryid)
bipolar_disorder_total_pt <- union(union(bipolar_disorder_indi_pt, bipolar_disorder_reac_pt), bipolar_disorder_drug)
## Disproportionality analysis ----------------------------------------------------------
restrictions <- list(
"raw" = as.list(Demo$primaryid),
"indi_pt" = as.list(bipolar_disorder_indi_pt),
"reac_pt" = as.list(bipolar_disorder_reac_pt),
"drug" = as.list(bipolar_disorder_drug),
"total" = as.list(bipolar_disorder_total_pt)
)
disproportionality_df <- data.table()
for (n in 1:length(restrictions)) {
t <- restrictions[n]
t_name <- names(t)
t_pids <- unlist(t)
df <- disproportionality_analysis(
drug_selected = drug_selected,
reac_selected = reac_selected,
temp_drug = Drug[role_cod %in% c("PS", "SS")],
restriction = t_pids
)[, nested := t_name]
disproportionality_df <- rbindlist(list(disproportionality_df, df), fill = TRUE)
}
## Definitions --------------------------------------------------------------------------
disproportionality_df
substance | event | D_E | D_nE | D | nD_E | E | nD_nE | ROR_median | ROR_lower | ROR_upper | IC_median | IC_lower | IC_upper | label_ROR | label_IC | Bonferroni | ROR_signal | IC_signal | nested |
---|---|---|---|---|---|---|---|---|---|---|---|---|---|---|---|---|---|---|---|
paracetamol | ICD | 962 | 341983 | 342945 | 71334 | 72296 | 17183644 | 0.67 | 0.63 | 0.72 | -0.56 | -0.66 | -0.48 | 0.67 (0.63-0.72) [962] | -0.56 (-0.66–0.48) [962] | FALSE | no SDR | no SDR | raw |
aripiprazole | ICD | 5208 | 76217 | 81425 | 67088 | 72296 | 17449410 | 17.77 | 17.25 | 18.30 | 3.95 | 3.91 | 3.99 | 17.77 (17.25-18.3) [5208] | 3.95 (3.91-3.99) [5208] | TRUE | weak SDR | SDR | raw |
TGAs | ICD | 5666 | 90313 | 95979 | 66630 | 72296 | 17435314 | 16.41 | 15.96 | 16.89 | 3.84 | 3.79 | 3.87 | 16.41 (15.96-16.89) [5666] | 3.84 (3.79-3.87) [5666] | TRUE | weak SDR | SDR | raw |
pramipexole | ICD | 2376 | 8721 | 11097 | 69920 | 72296 | 17516906 | 68.26 | 65.14 | 71.25 | 5.68 | 5.62 | 5.73 | 68.26 (65.14-71.25) [2376] | 5.68 (5.62-5.73) [2376] | TRUE | weak SDR | SDR | raw |
paracetamol | ICD | 17 | 473 | 490 | 2890 | 2907 | 72658 | 0.90 | 0.52 | 1.46 | -0.14 | -0.96 | 0.43 | 0.9 (0.52-1.46) [17] | -0.14 (-0.96-0.43) [17] | FALSE | no SDR | no SDR | indi_pt |
aripiprazole | ICD | 1310 | 8131 | 9441 | 1597 | 2907 | 65000 | 6.55 | 6.06 | 7.08 | 1.85 | 1.76 | 1.92 | 6.55 (6.06-7.08) [1310] | 1.85 (1.76-1.92) [1310] | TRUE | weak SDR | SDR | indi_pt |
TGAs | ICD | 1371 | 9580 | 10951 | 1536 | 2907 | 63551 | 5.92 | 5.48 | 6.39 | 1.71 | 1.62 | 1.77 | 5.92 (5.48-6.39) [1371] | 1.71 (1.62-1.77) [1371] | TRUE | weak SDR | SDR | indi_pt |
pramipexole | ICD | 7 | 49 | 56 | 2900 | 2907 | 73082 | 3.59 | 1.37 | 7.99 | 1.50 | 0.20 | 2.35 | 3.59 (1.37-7.99) [7] | 1.5 (0.2-2.35) [7] | TRUE | weak SDR | SDR | indi_pt |
paracetamol | ICD | 17 | 254 | 271 | 582 | 599 | 9017 | 1.03 | 0.59 | 1.70 | 0.04 | -0.77 | 0.61 | 1.03 (0.59-1.7) [17] | 0.04 (-0.77-0.61) [17] | FALSE | no SDR | no SDR | reac_pt |
aripiprazole | ICD | 40 | 281 | 321 | 559 | 599 | 8990 | 2.28 | 1.58 | 3.23 | 1.01 | 0.49 | 1.39 | 2.28 (1.58-3.23) [40] | 1.01 (0.49-1.39) [40] | TRUE | weak SDR | SDR | reac_pt |
TGAs | ICD | 47 | 318 | 365 | 552 | 599 | 8953 | 2.39 | 1.70 | 3.30 | 1.06 | 0.58 | 1.41 | 2.39 (1.7-3.3) [47] | 1.06 (0.58-1.41) [47] | TRUE | weak SDR | SDR | reac_pt |
pramipexole | ICD | 26 | 20 | 46 | 573 | 599 | 9251 | 20.98 | 11.18 | 39.88 | 3.00 | 2.35 | 3.47 | 20.98 (11.18-39.88) [26] | 3 (2.35-3.47) [26] | TRUE | weak SDR | SDR | reac_pt |
paracetamol | ICD | 14 | 957 | 971 | 1305 | 1319 | 43236 | 0.48 | 0.26 | 0.82 | -0.99 | -1.89 | -0.37 | 0.48 (0.26-0.82) [14] | -0.99 (-1.89–0.37) [14] | FALSE | no SDR | no SDR | drug |
aripiprazole | ICD | 351 | 3424 | 3775 | 968 | 1319 | 40769 | 4.31 | 3.79 | 4.90 | 1.67 | 1.50 | 1.80 | 4.31 (3.79-4.9) [351] | 1.67 (1.5-1.8) [351] | TRUE | weak SDR | SDR | drug |
TGAs | ICD | 374 | 3774 | 4148 | 945 | 1319 | 40419 | 4.23 | 3.73 | 4.80 | 1.63 | 1.46 | 1.75 | 4.23 (3.73-4.8) [374] | 1.63 (1.46-1.75) [374] | TRUE | weak SDR | SDR | drug |
pramipexole | ICD | 14 | 67 | 81 | 1305 | 1319 | 44126 | 7.06 | 3.65 | 12.72 | 2.34 | 1.44 | 2.96 | 7.06 (3.65-12.72) [14] | 2.34 (1.44-2.96) [14] | TRUE | weak SDR | SDR | drug |
paracetamol | ICD | 41 | 1598 | 1639 | 4245 | 4286 | 111477 | 0.67 | 0.48 | 0.91 | -0.55 | -1.07 | -0.17 | 0.67 (0.48-0.91) [41] | -0.55 (-1.07–0.17) [41] | FALSE | no SDR | no SDR | total |
aripiprazole | ICD | 1566 | 10649 | 12215 | 2720 | 4286 | 102426 | 5.53 | 5.18 | 5.91 | 1.81 | 1.72 | 1.87 | 5.53 (5.18-5.91) [1566] | 1.81 (1.72-1.87) [1566] | TRUE | weak SDR | SDR | total |
TGAs | ICD | 1645 | 12356 | 14001 | 2641 | 4286 | 100719 | 5.07 | 4.75 | 5.41 | 1.68 | 1.60 | 1.74 | 5.07 (4.75-5.41) [1645] | 1.68 (1.6-1.74) [1645] | TRUE | weak SDR | SDR | total |
pramipexole | ICD | 35 | 117 | 152 | 4251 | 4286 | 112958 | 7.94 | 5.27 | 11.70 | 2.55 | 1.98 | 2.95 | 7.94 (5.27-11.7) [35] | 2.55 (1.98-2.95) [35] | TRUE | weak SDR | SDR | total |
render_forest(disproportionality_df, row = "event", facet_v = "substance", nested = "nested")
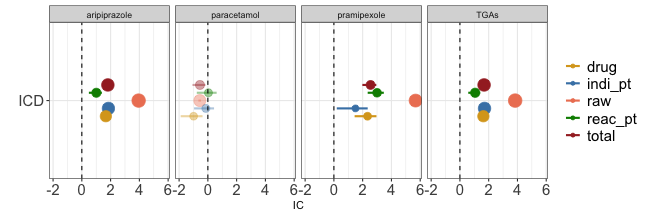
In this forest plot we see that in general, restricting to the confounder bipolar disorder, we see a smaller IC. Only paracetamol does not show a difference, as bipolar disorder configures more as a competitive exposure rather than a confounder (i.e., to be a confounder, a factor has to cause both the drug and the event, but bipolar disorder does not increase the chance of being prescribed paracetamol). We also see that the confidence interval is smaller when we manage to retrieve bigger background reference groups, and therefore using the information included in both Indi, Drug, and Reac is suggested.
We can also consider that bipolar disorder may have been reported using many other terms. How can we use the MedDRA HLGT “manic and bipolar mood disorders and disturbances” to make even bigger the reference group, still addressing the bias?
Note that this chunk of script works only if you have a MedDRA subscription and you have followed https://github.com/fusarolimichele/DiAna instructions to make your own DiAna-compatible MedDRA.
## Reference groups --------------------------------------------------------------------
# ....
import_MedDRA()
#> def soc
#> <char> <char>
#> 1: cong congenital, familial and genetic disorders
#> 2: inv investigations
#> 3: inv investigations
#> 4: inv investigations
#> 5: inv investigations
#> ---
#> 25408: inj&p injury, poisoning and procedural complications
#> 25409: preg pregnancy, puerperium and perinatal conditions
#> 25410: surg surgical and medical procedures
#> 25411: surg surgical and medical procedures
#> 25412: blood blood and lymphatic system disorders
#> hlgt
#> <char>
#> 1: metabolic and nutritional disorders congenital
#> 2: endocrine investigations (incl sex hormones)
#> 3: endocrine investigations (incl sex hormones)
#> 4: endocrine investigations (incl sex hormones)
#> 5: endocrine investigations (incl sex hormones)
#> ---
#> 25408: procedural related injuries and complications nec
#> 25409: pregnancy, labour, delivery and postpartum conditions
#> 25410: obstetric and gynaecological therapeutic procedures
#> 25411: male genital tract therapeutic procedures
#> 25412: white blood cell disorders
#> hlt
#> <char>
#> 1: inborn errors of steroid synthesis
#> 2: reproductive hormone analyses
#> 3: reproductive hormone analyses
#> 4: reproductive hormone analyses
#> 5: reproductive hormone analyses
#> ---
#> 25408: non-site specific procedural complications
#> 25409: normal pregnancy, labour and delivery
#> 25410: cervix therapeutic procedures
#> 25411: male genital tract therapeutic procedures nec
#> 25412: eosinophilic disorders
#> pt
#> <char>
#> 1: 11-beta-hydroxylase deficiency
#> 2: 17 ketosteroids urine
#> 3: 17 ketosteroids urine decreased
#> 4: 17 ketosteroids urine increased
#> 5: 17 ketosteroids urine normal
#> ---
#> 25408: incision site skin puckering
#> 25409: spontaneous rupture of membranes
#> 25410: cervix stent placement
#> 25411: sperm cryopreservation
#> 25412: paraneoplastic eosinophilia
bipolar_disorder_total_hlgt <- union(
union(
Indi[indi_pt %in% MedDRA[hlgt == "manic and bipolar mood disorders and disturbances"]$pt]$primaryid,
Reac[pt %in% MedDRA[hlgt == "manic and bipolar mood disorders and disturbances"]$pt]$primaryid
),
bipolar_disorder_drug
)
## Disproportionality analysis ----------------------------------------------------------
restrictions <- list(
"raw" = as.list(Demo$primaryid),
"total_pt" = as.list(bipolar_disorder_total_pt),
"total_hlgt" = as.list(bipolar_disorder_total_hlgt)
)
disproportionality_df <- data.table()
for (n in 1:length(restrictions)) {
t <- restrictions[n]
t_name <- names(t)
t_pids <- unlist(t)
df <- disproportionality_analysis(
drug_selected = drug_selected,
reac_selected = reac_selected,
temp_drug = Drug[role_cod %in% c("PS", "SS")],
restriction = t_pids
)[, nested := t_name]
disproportionality_df <- rbindlist(list(disproportionality_df, df), fill = TRUE)
}
## Definitions --------------------------------------------------------------------------
disproportionality_df
substance | event | D_E | D_nE | D | nD_E | E | nD_nE | ROR_median | ROR_lower | ROR_upper | IC_median | IC_lower | IC_upper | label_ROR | label_IC | Bonferroni | ROR_signal | IC_signal | nested |
---|---|---|---|---|---|---|---|---|---|---|---|---|---|---|---|---|---|---|---|
paracetamol | ICD | 962 | 341983 | 342945 | 71334 | 72296 | 17183644 | 0.67 | 0.63 | 0.72 | -0.56 | -0.66 | -0.48 | 0.67 (0.63-0.72) [962] | -0.56 (-0.66–0.48) [962] | FALSE | no SDR | no SDR | raw |
aripiprazole | ICD | 5208 | 76217 | 81425 | 67088 | 72296 | 17449410 | 17.77 | 17.25 | 18.30 | 3.95 | 3.91 | 3.99 | 17.77 (17.25-18.3) [5208] | 3.95 (3.91-3.99) [5208] | TRUE | weak SDR | SDR | raw |
TGAs | ICD | 5666 | 90313 | 95979 | 66630 | 72296 | 17435314 | 16.41 | 15.96 | 16.89 | 3.84 | 3.79 | 3.87 | 16.41 (15.96-16.89) [5666] | 3.84 (3.79-3.87) [5666] | TRUE | weak SDR | SDR | raw |
pramipexole | ICD | 2376 | 8721 | 11097 | 69920 | 72296 | 17516906 | 68.26 | 65.14 | 71.25 | 5.68 | 5.62 | 5.73 | 68.26 (65.14-71.25) [2376] | 5.68 (5.62-5.73) [2376] | TRUE | weak SDR | SDR | raw |
paracetamol | ICD | 41 | 1598 | 1639 | 4245 | 4286 | 111477 | 0.67 | 0.48 | 0.91 | -0.55 | -1.07 | -0.17 | 0.67 (0.48-0.91) [41] | -0.55 (-1.07–0.17) [41] | FALSE | no SDR | no SDR | total_pt |
aripiprazole | ICD | 1566 | 10649 | 12215 | 2720 | 4286 | 102426 | 5.53 | 5.18 | 5.91 | 1.81 | 1.72 | 1.87 | 5.53 (5.18-5.91) [1566] | 1.81 (1.72-1.87) [1566] | TRUE | weak SDR | SDR | total_pt |
TGAs | ICD | 1645 | 12356 | 14001 | 2641 | 4286 | 100719 | 5.07 | 4.75 | 5.41 | 1.68 | 1.60 | 1.74 | 5.07 (4.75-5.41) [1645] | 1.68 (1.6-1.74) [1645] | TRUE | weak SDR | SDR | total_pt |
pramipexole | ICD | 35 | 117 | 152 | 4251 | 4286 | 112958 | 7.94 | 5.27 | 11.70 | 2.55 | 1.98 | 2.95 | 7.94 (5.27-11.7) [35] | 2.55 (1.98-2.95) [35] | TRUE | weak SDR | SDR | total_pt |
paracetamol | ICD | 64 | 1791 | 1855 | 6243 | 6307 | 135909 | 0.77 | 0.59 | 0.99 | -0.35 | -0.76 | -0.05 | 0.77 (0.59-0.99) [64] | -0.35 (-0.76–0.05) [64] | FALSE | no SDR | no SDR | total_hlgt |
aripiprazole | ICD | 1776 | 13032 | 14808 | 4531 | 6307 | 124668 | 3.74 | 3.53 | 3.97 | 1.45 | 1.37 | 1.50 | 3.74 (3.53-3.97) [1776] | 1.45 (1.37-1.5) [1776] | TRUE | weak SDR | SDR | total_hlgt |
TGAs | ICD | 1890 | 15505 | 17395 | 4417 | 6307 | 122195 | 3.37 | 3.18 | 3.56 | 1.31 | 1.23 | 1.36 | 3.37 (3.18-3.56) [1890] | 1.31 (1.23-1.36) [1890] | TRUE | weak SDR | SDR | total_hlgt |
pramipexole | ICD | 104 | 196 | 300 | 6203 | 6307 | 137504 | 11.76 | 9.16 | 15.01 | 2.93 | 2.61 | 3.17 | 11.76 (9.16-15.01) [104] | 2.93 (2.61-3.17) [104] | TRUE | weak SDR | SDR | total_hlgt |
render_forest(disproportionality_df, row = "event", facet_v = "substance", nested = "nested")
#> Error in knitr::include_graphics("disproportionality_analysis-retrieve_with_MedDRA_forest-1.png", : Cannot find the file(s): "disproportionality_analysis-retrieve_with_MedDRA_forest-1.png"
Addressing notoriety bias
The FDA warning in 2016-03-05 concerning aripiprazole-induced impulsivity may have inflated the reporting distorting the results (i.e., notoriety bias). To address this bias we can restrict only reports submitted before the date of the warning, and repeat the disproportionality analysis. We compare this result with the raw analysis, the restriction to bipolar disorder, the restriction to bipolar disorder pre-warning, and finally this last complete restriction with also deduplication. All these analyses are performed considering only suspected drugs.
## Reference groups ---------------------------------------------------------------------
warning_date <- 20160305
pre_warning <- Demo[ifelse(is.na(init_fda_dt), fda_dt < warning_date, init_fda_dt < warning_date)]$primaryid
## Disproportionality analysis ----------------------------------------------------------
restrictions <- list(
"raw" = as.list(Demo$primaryid),
"indication_bias" = as.list(bipolar_disorder_total_pt),
"notoriety_bias" = as.list(pre_warning),
"both_biases" = as.list(intersect(
bipolar_disorder_total_pt,
pre_warning
)),
"both_biases_dedup" = as.list(intersect(
intersect(
bipolar_disorder_total_pt, pre_warning
),
Demo[RB_duplicates == FALSE]$primaryid
))
)
disproportionality_df <- data.table()
for (n in 1:length(restrictions)) {
t <- restrictions[n]
t_name <- names(t)
t_pids <- unlist(t)
df <- disproportionality_analysis(
drug_selected = drug_selected,
reac_selected = reac_selected,
temp_drug = Drug[role_cod %in% c("PS", "SS")],
restriction = t_pids
)[, nested := t_name]
disproportionality_df <- rbindlist(list(disproportionality_df, df), fill = TRUE)
}
## Definitions --------------------------------------------------------------------------
disproportionality_df
substance | event | D_E | D_nE | D | nD_E | E | nD_nE | ROR_median | ROR_lower | ROR_upper | IC_median | IC_lower | IC_upper | label_ROR | label_IC | Bonferroni | ROR_signal | IC_signal | nested |
---|---|---|---|---|---|---|---|---|---|---|---|---|---|---|---|---|---|---|---|
paracetamol | ICD | 962 | 341983 | 342945 | 71334 | 72296 | 17183644 | 0.67 | 0.63 | 0.72 | -0.56 | -0.66 | -0.48 | 0.67 (0.63-0.72) [962] | -0.56 (-0.66–0.48) [962] | FALSE | no SDR | no SDR | raw |
aripiprazole | ICD | 5208 | 76217 | 81425 | 67088 | 72296 | 17449410 | 17.77 | 17.25 | 18.30 | 3.95 | 3.91 | 3.99 | 17.77 (17.25-18.3) [5208] | 3.95 (3.91-3.99) [5208] | TRUE | weak SDR | SDR | raw |
TGAs | ICD | 5666 | 90313 | 95979 | 66630 | 72296 | 17435314 | 16.41 | 15.96 | 16.89 | 3.84 | 3.79 | 3.87 | 16.41 (15.96-16.89) [5666] | 3.84 (3.79-3.87) [5666] | TRUE | weak SDR | SDR | raw |
pramipexole | ICD | 2376 | 8721 | 11097 | 69920 | 72296 | 17516906 | 68.26 | 65.14 | 71.25 | 5.68 | 5.62 | 5.73 | 68.26 (65.14-71.25) [2376] | 5.68 (5.62-5.73) [2376] | TRUE | weak SDR | SDR | raw |
paracetamol | ICD | 41 | 1598 | 1639 | 4245 | 4286 | 111477 | 0.67 | 0.48 | 0.91 | -0.55 | -1.07 | -0.17 | 0.67 (0.48-0.91) [41] | -0.55 (-1.07–0.17) [41] | FALSE | no SDR | no SDR | indication_bias |
aripiprazole | ICD | 1566 | 10649 | 12215 | 2720 | 4286 | 102426 | 5.53 | 5.18 | 5.91 | 1.81 | 1.72 | 1.87 | 5.53 (5.18-5.91) [1566] | 1.81 (1.72-1.87) [1566] | TRUE | weak SDR | SDR | indication_bias |
TGAs | ICD | 1645 | 12356 | 14001 | 2641 | 4286 | 100719 | 5.07 | 4.75 | 5.41 | 1.68 | 1.60 | 1.74 | 5.07 (4.75-5.41) [1645] | 1.68 (1.6-1.74) [1645] | TRUE | weak SDR | SDR | indication_bias |
pramipexole | ICD | 35 | 117 | 152 | 4251 | 4286 | 112958 | 7.94 | 5.27 | 11.70 | 2.55 | 1.98 | 2.95 | 7.94 (5.27-11.7) [35] | 2.55 (1.98-2.95) [35] | TRUE | weak SDR | SDR | indication_bias |
paracetamol | ICD | 415 | 79782 | 80197 | 37167 | 37582 | 6296895 | 0.88 | 0.79 | 0.97 | -0.18 | -0.35 | -0.07 | 0.88 (0.79-0.97) [415] | -0.18 (-0.35–0.07) [415] | FALSE | no SDR | no SDR | notoriety_bias |
aripiprazole | ICD | 1062 | 31287 | 32349 | 36520 | 37582 | 6345390 | 5.89 | 5.53 | 6.27 | 2.48 | 2.38 | 2.55 | 5.89 (5.53-6.27) [1062] | 2.48 (2.38-2.55) [1062] | TRUE | weak SDR | SDR | notoriety_bias |
TGAs | ICD | 1081 | 31812 | 32893 | 36501 | 37582 | 6344865 | 5.90 | 5.55 | 6.28 | 2.48 | 2.38 | 2.55 | 5.9 (5.55-6.28) [1081] | 2.48 (2.38-2.55) [1081] | TRUE | weak SDR | SDR | notoriety_bias |
pramipexole | ICD | 1846 | 4831 | 6677 | 35736 | 37582 | 6371846 | 68.14 | 64.42 | 72.04 | 5.54 | 5.46 | 5.59 | 68.14 (64.42-72.04) [1846] | 5.54 (5.46-5.59) [1846] | TRUE | weak SDR | SDR | notoriety_bias |
paracetamol | ICD | 17 | 573 | 590 | 1914 | 1931 | 60406 | 0.93 | 0.54 | 1.51 | -0.09 | -0.91 | 0.47 | 0.93 (0.54-1.51) [17] | -0.09 (-0.91-0.47) [17] | FALSE | no SDR | no SDR | both_biases |
aripiprazole | ICD | 198 | 4771 | 4969 | 1733 | 1931 | 56208 | 1.34 | 1.15 | 1.56 | 0.37 | 0.14 | 0.54 | 1.34 (1.15-1.56) [198] | 0.37 (0.14-0.54) [198] | TRUE | weak SDR | SDR | both_biases |
TGAs | ICD | 201 | 4809 | 5010 | 1730 | 1931 | 56170 | 1.35 | 1.16 | 1.57 | 0.38 | 0.15 | 0.55 | 1.35 (1.16-1.57) [201] | 0.38 (0.15-0.55) [201] | TRUE | weak SDR | SDR | both_biases |
pramipexole | ICD | 25 | 63 | 88 | 1906 | 1931 | 60916 | 12.68 | 7.62 | 20.50 | 2.99 | 2.32 | 3.46 | 12.68 (7.62-20.5) [25] | 2.99 (2.32-3.46) [25] | TRUE | weak SDR | SDR | both_biases |
paracetamol | ICD | 17 | 547 | 564 | 1889 | 1906 | 57300 | 0.94 | 0.54 | 1.52 | -0.08 | -0.90 | 0.48 | 0.94 (0.54-1.52) [17] | -0.08 (-0.9-0.48) [17] | FALSE | no SDR | no SDR | both_biases_dedup |
aripiprazole | ICD | 188 | 4194 | 4382 | 1718 | 1906 | 53653 | 1.39 | 1.19 | 1.63 | 0.42 | 0.18 | 0.60 | 1.39 (1.19-1.63) [188] | 0.42 (0.18-0.6) [188] | TRUE | weak SDR | SDR | both_biases_dedup |
TGAs | ICD | 191 | 4231 | 4422 | 1715 | 1906 | 53616 | 1.41 | 1.20 | 1.64 | 0.43 | 0.19 | 0.60 | 1.41 (1.2-1.64) [191] | 0.43 (0.19-0.6) [191] | TRUE | weak SDR | SDR | both_biases_dedup |
pramipexole | ICD | 25 | 63 | 88 | 1881 | 1906 | 57784 | 12.19 | 7.32 | 19.69 | 2.94 | 2.27 | 3.41 | 12.19 (7.32-19.69) [25] | 2.94 (2.27-3.41) [25] | TRUE | weak SDR | SDR | both_biases_dedup |
render_forest(disproportionality_df, row = "event", facet_v = "substance", nested = "nested")
#> Error in knitr::include_graphics("disproportionality_analysis-notoriety_bias_forest-1.png", : Cannot find the file(s): "disproportionality_analysis-notoriety_bias_forest-1.png"
Here you see in green the results of the analysis restricting to the pre-warning. You can see that this analysis does not affect bipolar disorder nor headache, but it affects all the impulse control disorders apart gambling. Plausibly the FDA warning was based mainly on suspect aripiprazole-induced gambling disorder.
Therefore, you can see that even before the warning and taking into account all the considered biases, the FDA had enough information to publish the warning.
Disproportionality trend
Can we check when exactly a signal appeared in the FAERS? For example in the case of the disproportionality restricted to bipolar disorder and suspected drugs only?
For this inquiry, DiAna proposes a specific function: disproportionality_trend, which allow to adopt a granularity of a month, a quarter, or a year.
## Import data --------------------------------------------------------------------------
import("DEMO_SUPP")
#> primaryid rpsr_cod caseid caseversion i_f_cod auth_num e_sub
#> <num> <fctr> <int> <fctr> <fctr> <char> <fctr>
#> 1: 45217461 HP 4521746 1 I <NA> N
#> 2: 57910401 <NA> 5791040 1 I <NA> N
#> 3: 56962401 <NA> 5696240 1 I <NA> N
#> 4: 54662121 FGN 5466212 1 I <NA> N
#> 5: 54662121 HP 5466212 1 I <NA> N
#> ---
#> 18283734: 236937711 CSM 23693771 1 I 697702 N
#> 18283735: 236937721 CSM 23693772 1 I 697662 N
#> 18283736: 236937741 CSM 23693774 1 I 697678 N
#> 18283737: 237079261 CSM 23707926 1 I 697660 N
#> 18283738: 237079291 CSM 23707929 1 I 697693 N
#> lit_ref rept_dt to_mfr mfr_sndr mfr_num mfr_dt
#> <char> <int> <char> <char> <char> <int>
#> 1: <NA> NA <NA> <NA> <NA> NA
#> 2: <NA> NA <NA> <NA> <NA> NA
#> 3: <NA> NA <NA> <NA> <NA> NA
#> 4: <NA> 19961015 <NA> ELI LILLY AND CO ZA96101984A 19961010
#> 5: <NA> 19961015 <NA> ELI LILLY AND CO ZA96101984A 19961010
#> ---
#> 18283734: <NA> 20240331 N FDA-CTU <NA> 20240331
#> 18283735: <NA> 20240331 N FDA-CTU <NA> 20240331
#> 18283736: <NA> 20240331 <NA> FDA-CTU <NA> 20240331
#> 18283737: <NA> 20240331 Y FDA-CTU <NA> 20240331
#> 18283738: <NA> 20240331 Y FDA-CTU <NA> 20240331
#> quarter
#> <fctr>
#> 1: 20Q3
#> 2: 20Q3
#> 3: 20Q3
#> 4: 20Q3
#> 5: 20Q3
#> ---
#> 18283734: 24Q1
#> 18283735: 24Q1
#> 18283736: 24Q1
#> 18283737: 24Q1
#> 18283738: 24Q1
## Disproportionality analysis ----------------------------------------------------------
disproportionality_trend_df <- disproportionality_trend(
drug_selected = "brexpiprazole",
reac_selected = unlist(reac_selected),
temp_drug = Drug[role_cod %in% c("PS", "SS")],
time_granularity = "year"
)
plot_disproportionality_trend(disproportionality_trend_results = disproportionality_trend_df)
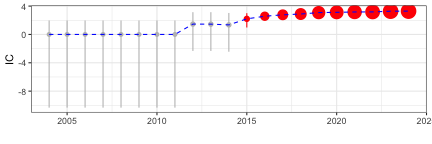
Comparing several disproportionality metrics
The ROR and the IC, while extremely common in pharmacovigilance, are not the only disproportionality metrics. To compare their results with other disproportionality metrics you can use the disproportionality_comparison function
disproportionality_comparison(
drug_count = length(unique(Drug[substance == "aripiprazole" & role_cod %in% c("PS", "SS")]$primaryid)),
event_count = length(unique(Reac[pt == "impulsive behaviour"]$primaryid)),
drug_event_count = length(intersect(Drug[substance == "aripiprazole" & role_cod %in% c("PS", "SS")]$primaryid, Reac[pt == "impulsive behaviour"]$primaryid)),
tot = nrow(Demo)
)
#> E nE
#> D 379 81046
#> nD 2885 17514169
#>
#>
#> ROR = 28.39 (25.4-31.58)
#> PRR = 28.26 (25.4-31.45)
#> RRR = 25.1 (22.57-27.91)
#> IC = 4.6 (4.43-4.72)
#> IC_gamma = 4.48 (4.46-4.75)